Best Data Versioning Tools for MLOps
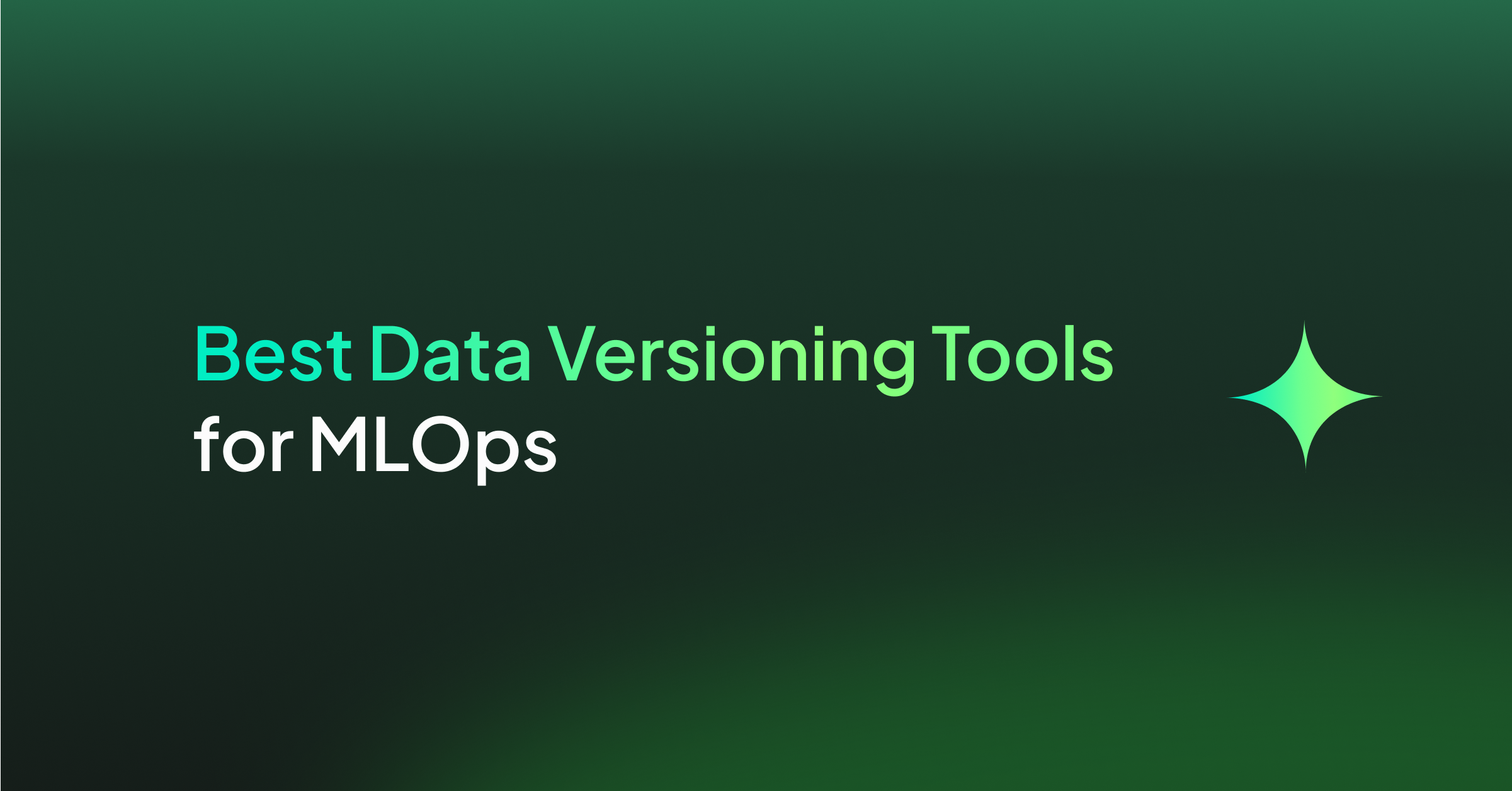
How does your team keep track of all your data for your machine learning models and experiments? This is a common issue that pops up for data science teams. To keep up-to-date and aligned, following all version updates, your team needs the right tools. Take a look below to see a list of top data versioning tools in the MLOps space.
What are Data Versioning Tools and Why Are They Important?
Data versioning tools can help you build a repository for your data, track experiments and model lineage, reduce errors, and improve workflows and collaboration with your team. These tools can be extremely helpful for organizing data version control and enabling easy reproducibility of your machine learning models.
The following list highlights useful Data Versioning management tools and their specific benefits.
1. DAGsHub
DAGsHub enables data scientists and ML engineers to work together efficiently. It integrates open-source tools like Git, DVC, MLflow, and Jenkins so that you can track and version code, data, models, pipelines, and experiments in one place.
Benefits:
- Your project in one place: Manage your code, notebooks, data, models, pipelines, and experiments and easily connect to plugins for automation, all with open source tools and open formats
- Zero configuration: Each project comes with a free, built-in DVC data storage and MLflow server, with team access controls
- Diff, compare, and review anything: Allows you to have different Jupyter notebooks, tables, images, experiments, and even MRI data, so you can compare apples to apples, review, and make sense of your work
- Reproducibility is a click away: Get all components of an experiment on your system
2. DVC
DVC is an open-source tool for data science and machine learning projects, used to replace spreadsheet and document sharing tools. It replaces both ad-hoc scripts for tracking, moving, and deploying different model versions, in addition to ad-hoc data file suffixes and prefixes.
Benefits:
- Simple command line Git-like experience
- Doesn’t require maintaining or installing databases
- Doesn’t depend on proprietary online services
- Management and versioning of datasets and machine learning models
- Saves data in S3, Google cloud, Azure, Alibaba cloud, SSH server, HDFS, or even local HDD RAID
- Makes projects reproducible, shareable, and helps to answer questions about how a model was built
- Assists in managing experiments with Git tags/branches and tracking metrics
3. Pachyderm
Pachyderm is a tool for data scientists to use for version-controlled, automated, end-to-end data pipelines.
Benefits:
- Containerized: built on Docker and Kubernetes
- Can run whatever languages or libraries your pipeline needs, easily deploying them on any cloud provider or on prem
- Version Control: version controls your data as it’s processed
- Can always ask the system how data has changed, see differences, and revert
- Provenance (aka data lineage): tracks where data comes from
- Keeps track of all the code and data that created a result
- Parallelization: can efficiently schedule massively parallel workloads
- Incremental Processing: understands how your data has changed and is smart enough to only process the new data
4. lakeFS
An open-source data lake management platform that transforms your object storage into a Git-like repository. It enables you to manage your data lake the way you would your code and run parallel pipelines for experimentation and CI/CD for your data.
Benefits:
- Scalable: Version control data at exabyte scale
- Flexible: Run git operations like branch, commit, and merge over your data in any storage service
- Develop Faster: Zero copy branching for frictionless experimentation, easy collaboration
- Enable Clean Workflows: Use pre-commit & merge hooks for CI/CD workflows
- Resilient: Recover from data issues faster with revert capability
Find the Right MLOps Tools for Your Needs
In recent years the MLOps space is continuing to grow with more tools that are designed to make model building and training simpler, more automated and scalable. However, it’s not always easy to determine which MLOps tools answer your needs best.
Building an ML infrastructure requires a number of MLOps tools for data versioning, training orchestration, feature store, model serving, experiment tracking, model monitoring, and explainability. But finding the right tools is a project in itself. To make this process easier, we created MLOps.toys – a curated list of useful MLOps tools – we welcome you to take a look and explore 🙂