Credit Risk Monitoring: The Basics and AI/ML Techniques
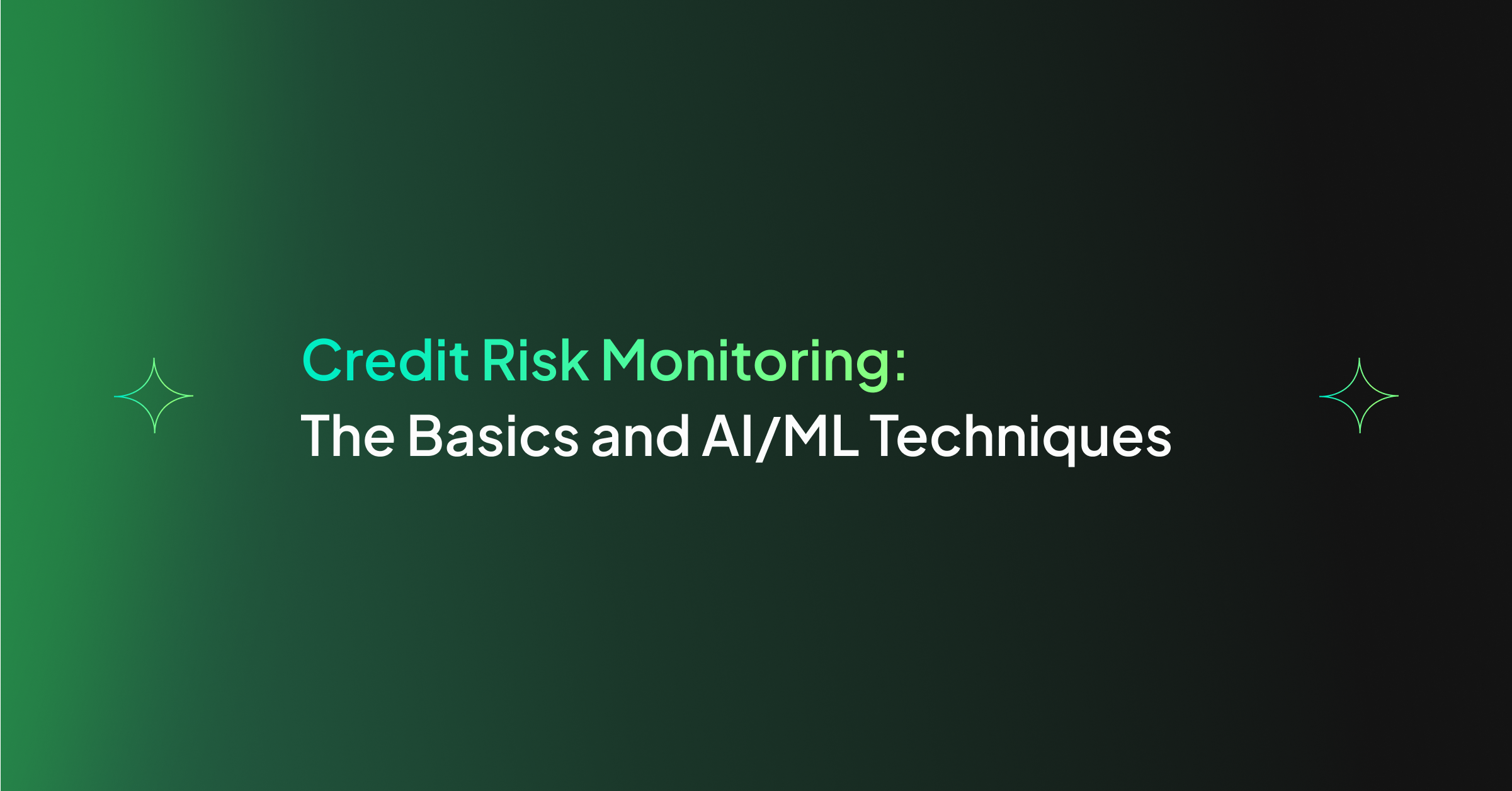
What Is Credit Risk Monitoring?
Credit risk monitoring is the process of continuously evaluating and monitoring the creditworthiness of borrowers, to determine the risk of default and ensure that the loan agreements are being adhered to. This helps financial institutions make informed lending decisions and take appropriate action to manage potential losses. It involves collecting, analyzing, and interpreting financial and non-financial data to assess a borrower’s ability and willingness to repay debt obligations.
Why Is Credit Risk Monitoring Important?
Credit risk monitoring is an important component of a lender’s risk management strategy because it helps to minimize the risk of loan losses. By regularly assessing the creditworthiness of potential and existing clients, lenders can identify and manage potential risks before they become significant problems. This helps to ensure that a lender’s lending portfolio remains profitable and reduces the likelihood of financial losses.
Additionally, credit risk monitoring helps lenders to maintain good relationships with their clients. By staying informed about their financial health, lenders can work with clients to address any issues and develop solutions to help them maintain good credit standing. This can help to build trust and credibility between the lender and the client, which is important for long-term business relationships.
Credit risk monitoring also helps lenders to comply with regulatory requirements. In many jurisdictions, lenders are required to have systems in place to monitor credit risk and to report any potential issues to regulatory authorities. This helps to maintain stability in the financial system and to protect consumers from harmful lending practices.
Credit Risk Monitoring Techniques
Credit risk monitoring techniques are methods used by lenders to assess and manage the credit risk posed by potential and existing clients. Some of the most commonly used credit risk monitoring techniques include:
- Financial statement analysis: This involves reviewing a client’s financial statements, such as balance sheets, income statements, and cash flow statements, to assess their financial health and creditworthiness. Lenders look for key financial metrics, such as revenue, expenses, and debt levels, to determine if a client has the ability to repay a loan.
- Credit scoring: This is a statistical method of evaluating a client’s credit risk based on a numerical score. Credit scores are calculated using information from credit reports, such as payment history, debt levels, and length of credit history. The score provides a quick and objective assessment of a client’s credit risk, which can be used to make lending decisions.
- Stress testing: This is a simulation technique used to assess the impact of adverse economic conditions on a client’s ability to repay a loan. Stress testing helps lenders to determine the impact of events such as changes in interest rates, economic downturns, or unexpected expenses on a client’s financial health.
- Collateral management: This involves the use of assets, such as property or equipment, to secure a loan. Lenders monitor the value of the collateral over time to ensure that it continues to provide sufficient security for the loan. If the value of the collateral decreases, the lender may require additional security to maintain the loan.
AI and Machine Learning for Credit Risk Monitoring
Monitoring and Collections
AI and machine learning can be used to improve credit risk monitoring and collections efforts in several ways, including:
- Targeted collections: By analyzing historical data on collections efforts, machine learning algorithms can determine which customers are most likely to repay and which customers are most likely to default. This enables collections teams to prioritize their efforts and focus on the customers with the highest probability of successful recovery.
- Automated collections: AI and machine learning can automate collections efforts, allowing financial institutions to reduce costs and increase efficiency. For example, AI chatbots can be used to engage with customers and collect payments, while machine learning algorithms can be used to determine the best time and method for reaching out to customers.
Looking For Early Warning Signals
AI and machine learning can be used to identify early warning signals of credit risk. By analyzing large volumes of data, such as transactional data, credit scores, and other financial indicators, these algorithms can identify patterns and trends that may indicate increased credit risk. Here are some examples:
- Changes in spending habits: AI can help detect changes in a customer’s spending habits. For example, if a customer suddenly starts spending more than they typically do, this could be an indication that they are experiencing financial difficulties and may be at risk of default.
- Indicators of risk: Machine learning algorithms can detect behaviors that could indicate the customer is experiencing financial difficulties and may be at risk of default. For example, when a customer is consistently making late payments, utilizing a higher percentage of their available credit, a sudden drop in the customer’s credit score, or a change in employment status.
By identifying these and other early warning signals of credit risk, financial institutions can take action before the risk becomes a problem. For example, they may adjust credit limits, increase collections efforts, or modify the loan terms to reduce the risk of default.
Credit Risk Analysis
AI and machine learning algorithms can analyze large volumes of transactional data and other financial indicators, and identify patterns and anomalies that may indicate fraudulent activity. Here are some examples:
- Network analysis: Machine learning algorithms can analyze the relationships between customers, accounts, and transactions to identify suspicious patterns of activity, such as customers who are linked to multiple accounts with suspicious transactions.
- Behavioral biometrics: AI can analyze a customer’s behavioral biometrics, such as their typing speed, mouse movements, and other patterns of behavior. This can help identify when a customer’s account is being used by someone else, such as a fraudster.
- Predictive models: These models are trained on historical data to identify patterns and trends that may indicate fraud. Once trained, the models can be used to predict the likelihood of fraud for new transactions.
These advanced technologies can help detect fraudulent activity faster and more accurately than traditional methods, enabling financial institutions to take action to prevent or mitigate losses.
Challenges of ML Credit Risk Monitoring
Machine learning is a powerful tool that can be used to improve credit risk monitoring and prediction, but it also presents some challenges. Here are some of the challenges of machine learning with credit risk monitoring:
- Data quality: Machine learning models are only as good as the data they are trained on. If the data is incomplete, inaccurate, or biased, the model’s predictions will also be inaccurate or biased. In the case of credit risk monitoring, it can be difficult to obtain high-quality data, as credit history information can be incomplete or out of date.
- Model complexity: Machine learning models can be complex, and it can be difficult to understand how they are making predictions. This can be a challenge for credit risk monitoring, as lenders need to be able to explain their lending decisions to regulators, auditors, and customers.
- Interpretability: Related to the previous challenge, it can be difficult to interpret the results of a machine learning model, particularly if the model is making predictions based on a large number of variables. This can make it difficult to identify which variables are most important in determining credit risk.
- Regulatory compliance: Credit risk monitoring is subject to a variety of regulations, and machine learning models may need to comply with specific regulatory requirements. For example, regulators may require that lenders explain how a machine learning model is making its predictions, or that the model is not biased against certain groups of borrowers.
- Ethical concerns: Machine learning models can be biased, particularly if they are trained on biased data. This can be a concern in credit risk monitoring, as biased models could result in discriminatory lending practices. Lenders need to be aware of the potential for bias and take steps to mitigate it.