Data Drift: Types, Detection Methods, and Mitigation
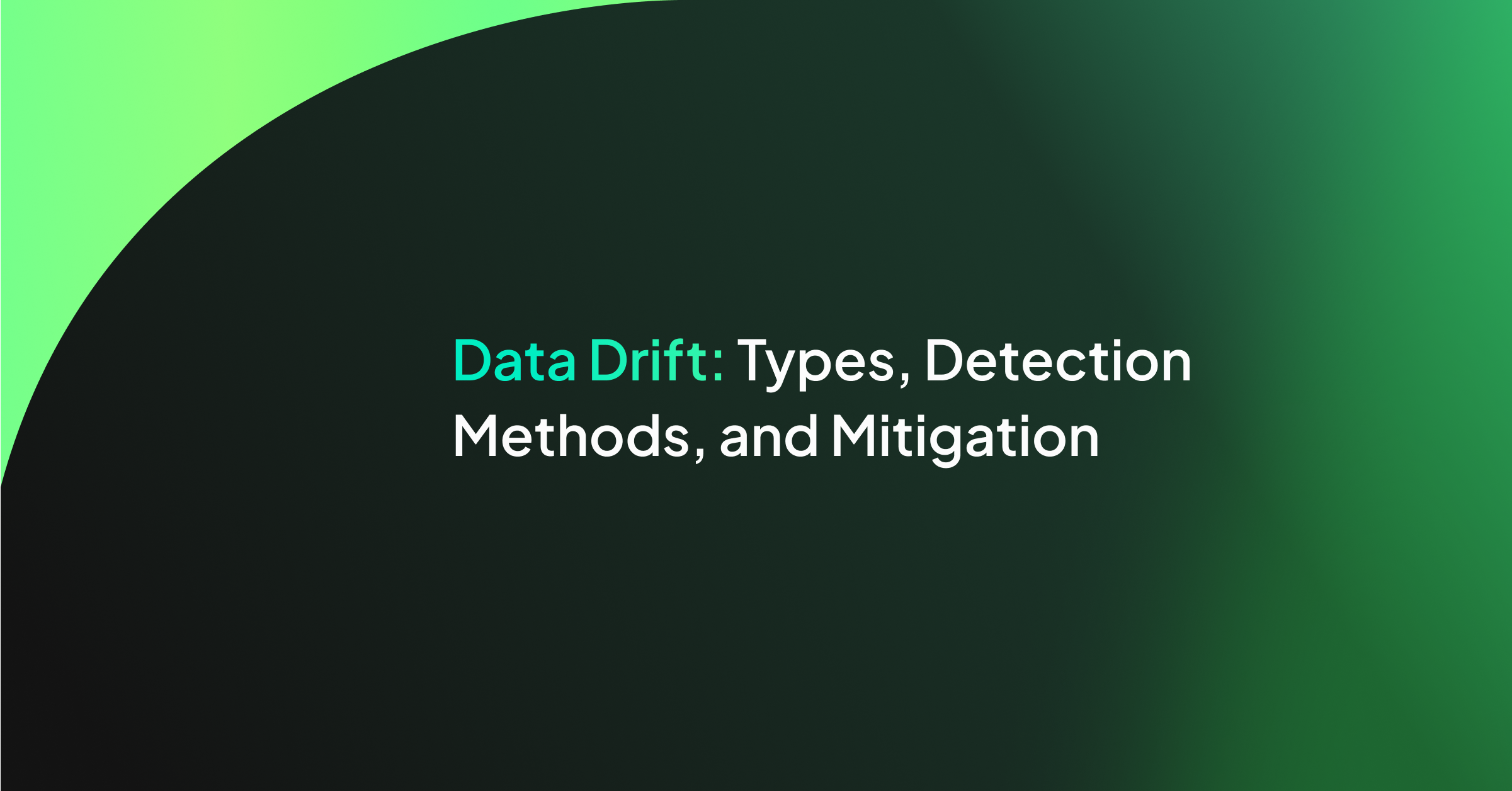
What Is Data Drift?
Machine learning models are only as good as the data they ingest during and after training. Data drift refers to a change in the distribution of a model’s input data over time. In other words, it refers to a situation where the input data that a machine learning model was trained on no longer accurately represents the data that the model is being applied to.
Data drift can have a significant impact on the performance of machine learning models, as a model that was trained on a different distribution of data may not be able to accurately predict or classify new data. This can cause a model to become less accurate over time, or even lead to the model’s performance degrading rapidly.
It’s important to keep an eye on the performance of the model over time and keep track of any changes in the input data, so that data drift can be identified and addressed as soon as possible.
This is part of an extensive series of guides about machine learning.
Types of Data Drift
Concept Drift
Concept drift is when the relationship between the inputs and outputs of a machine learning model changes in the real world, compared to those relations when the model was trained. In other words, predictions generated by the model for certain inputs, which used to be correct, are no longer relevant.
For example, a model that was trained to detect fraudulent credit card transactions may become less accurate over time as criminals change their tactics. This is the most basic form of data drift.
Learn more in our detailed guide to concept drift
Covariate Shift
Covariate shift is similar to concept drift, but it is a more severe problem. In covariate drift, not only is there a shift in the relation between inputs and outputs, but in addition, the input data changes.
For example, a model that was trained on data from a specific geographical region may become less accurate when applied to data from a different region due to different cultural influences or purchasing habits. Here there is a change in the way the model needs to analyze inputs, and the inputs themselves are also different.
Prior Probability Shift
Prior probability shift occurs when the proportion of the different classes in the data changes over time. For example, if a binary classification model was trained to detect spam email, and the proportion of spam email in the population changes, the model’s performance may suffer as its prior probability assumptions are not accurate anymore.
Methods for Detecting Data Drift
Population Stability Index
The PSI is a measure of the change in the distribution of a feature between the training and test data. It is calculated as the difference in the cumulative probability of a feature between the two datasets. A high PSI value indicates a significant change in the distribution of the feature, which may indicate data drift.
The formula to calculate PSI looks like this:
PSI = ((Actual% – Expected%) * ln(Actual% * Expected%))
Kolmogorov-Smirnov
The Kolmogorov-Smirnov test is a non-parametric test that can be used to determine whether two samples come from the same distribution. This test can be used to detect data drift by comparing the distribution of the training data and the distribution of the test data.
The formula looks like this:
Dn,m = supx|F1,n(x) – F2,m(x)| Fn(x) = 12i=1nI[-,x](Xi)
F1,n(x) is the distribution function for previous data (n), while F2,m(x) is the distribution function for new data (m), and supx refers to the subset of x samples that maximizes the two functions.
Kullback-Leibler Divergence
KL divergence is a measure of the difference between two probability distributions. It can be used to detect data drift by comparing the distribution of the training data and the distribution of the test data.
Here is an example of the KL divergence formula with A and B representing the old and new data distributions, respectively:
KL(A||B) = – xB(x) * logA(x)B(x)
The divergence can be anything between 0 and infinity – score of 0 means the distributions are identical.
Jensen-Shannon Divergence
JS divergence is a symmetric version of the KL divergence method, which can be used to detect the similarity or dissimilarity between two probability distributions. Following is the formula used in JS divergence:
JS(B||A) = 12(KL(B||M) + KL(A||M))
Learn more in our detailed guide to data drift detection (coming soon)
Overcoming Drift in Machine Learning
Here are a few strategies that can be used to solve data drift:
- Continuous monitoring: One effective approach to addressing data drift is to continuously monitor production inferences generated by the model, as compared to training data, to detect changes as they occur. This can be done using statistical tests or data quality checks to identify any unusual patterns or changes in the data. By regularly monitoring the data, organizations can quickly detect and address data drift, helping to ensure that the machine learning model remains accurate and continues to perform well.
- Data cleansing: Data cleansing is the process of identifying and correcting errors and inconsistencies in data. By regularly cleaning the data used to train machine learning models, organizations can help to reduce the effects of data drift. This can involve techniques such as deduplication, standardization, and validation to ensure that the data is accurate and consistent.
- Retraining: If data drift is detected, it may be necessary to retrain the machine learning model on a new data set to ensure that it remains accurate. This can be done on a regular schedule, such as monthly or quarterly, or as needed in response to detected data drift. When retraining the model, it is important to use a representative sample of the current data to ensure that the model accurately reflects the current characteristics of the data.
- Ensemble models: Ensemble models are machine learning models that are built by combining the predictions of multiple models. Because ensemble models are less sensitive to changes in individual models, they can be more robust to data drift. By using an ensemble of models, organizations can mitigate the effects of data drift and improve the accuracy of their machine learning models.
- Data augmentation: Data augmentation is the process of generating new data samples by making small variations to existing data. By using data augmentation to generate additional training data, organizations can help to reduce the effects of data drift. This can be particularly useful in cases where there is a limited amount of training data available, as it allows organizations to create a larger and more diverse data set to train the machine learning model on.
By implementing these strategies, organizations can effectively address data drift and ensure that their machine learning models continue to perform well over time.
Learn more in our detailed guides to:
- Data drift in machine learning (coming soon)
- Concept drift vs. data drift (coming soon)
Tracking Data Drift
By identifying and addressing data drift early on, businesses can avoid the negative consequences of inaccurate predictions, such as lost revenue, reduced customer satisfaction, and increased operational costs. Thus, monitoring ML models for data drift is crucial for maintaining business continuity and maximizing the benefits of machine learning.