The GenAI Chasm: What Is It & How to Cross It
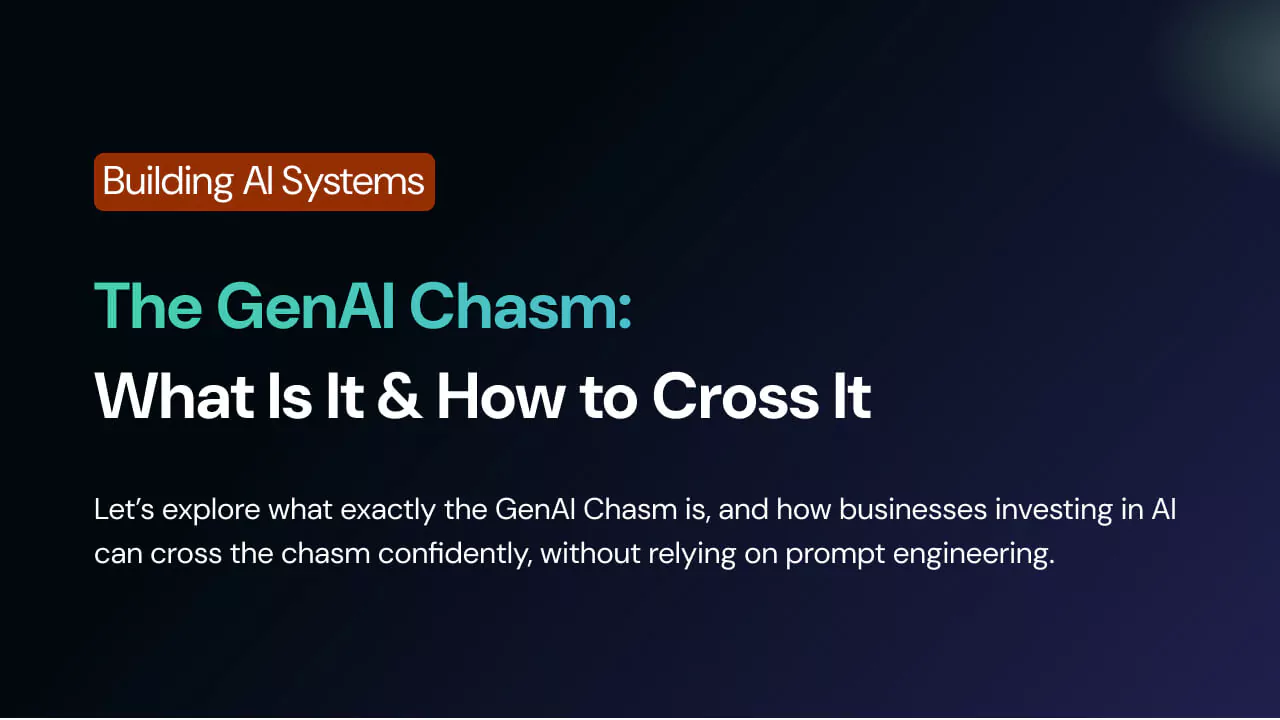
As AI technology advances and becomes increasingly used amongst businesses, there are certain issues that have arisen that are now coming to light, one of them being the GenAI Chasm. Let’s explore what exactly the GenAI Chasm is, and how businesses investing in AI can cross the chasm confidently, without relying on prompt engineering.
What is the GenAI Chasm?
Liran Hason, VP of AI at Coralogix coined this term after having over 2 years of experience speaking to potential customers about their GenAI products. He noticed that all businesses trying to implement AI apps struggle to get past the pilot phase, known as the chasm.
Here is an example of business ZYX that tries to cross the GenAI chasm:
- ZYX decision-makers map out over 200 AI app ideas that benefit internal and external businesses. These include IT support chatbots, documentation summary apps, consumer-facing customer service bots, and more.
- Due to limited manpower in the development team, they decide to work on only 15 of the most important apps initially.
- The 15 apps are sent to the pilot phase to test whether the app is working correctly and not misbehaving.
- Out of the 15 apps, 14 are found to be largely misbehaving, and only 1 is deemed ready to go live
Why Do Over 91% of Apps Not Make it Out of the Pilot Phase?
Hallucinations, prompt injection risks, compliance issues, and unintended behavior are some of the main reasons that only a small percentage of apps can actually go live. Releasing an app with these issues risks damaging brand reputation, exposing sensitive information, and losing customer trust.
GenAI is an incredible tool that businesses can use to enhance their productivity and engagement with customers. However, when presenting hallucinations and incorrect behavior, most of these apps will never go live. Crossing this chasm to get AI apps to go live is a difficulty almost every business investing in AI is struggling with, but there is a solution to this situation.
How to Cross the GenAI Chasm Confidently
One proven way to help businesses cross the chasm and release more AI apps with confidence is by implementing evaluators that sit between the LLM and the user. AI evaluators that can vet every response that comes in from the user, and that goes out from the LLM passes through these evaluators, ensuring that you are alerted to hallucinations, prompt injections, and inappropriate behavior in real-time.
Why is Prompt Engineering Not the Ultimate Solution to Solving Hallucinations
While prompt engineering is currently the preferred method of mitigating hallucinations, it is not the ultimate solution that provides long-term results in the app. Studies have shown that adding more words to the system prompt decreases accuracy, making it more susceptible to hallucinations. So using prompt engineering to catch inappropriate behavior and incorrect results can only further worsen this issue as a result.
App Accuracy Decreases as More Tokens Added
Coralogix’s Evaluators – the Fastest, Most Effective Solution to Crossing the Chasm
Evaluators is the preferred method to use when crossing the GenAI chasm. These evaluators provide out-of-the-box policies to alert you to hallucinations and inappropriate LLM behavior. Simply integrate Coralogix’s AI observability solution and safeguard your app in a few minutes.