Understanding Why AI Guardrails Are Necessary: Ensuring Ethical and Responsible AI Use
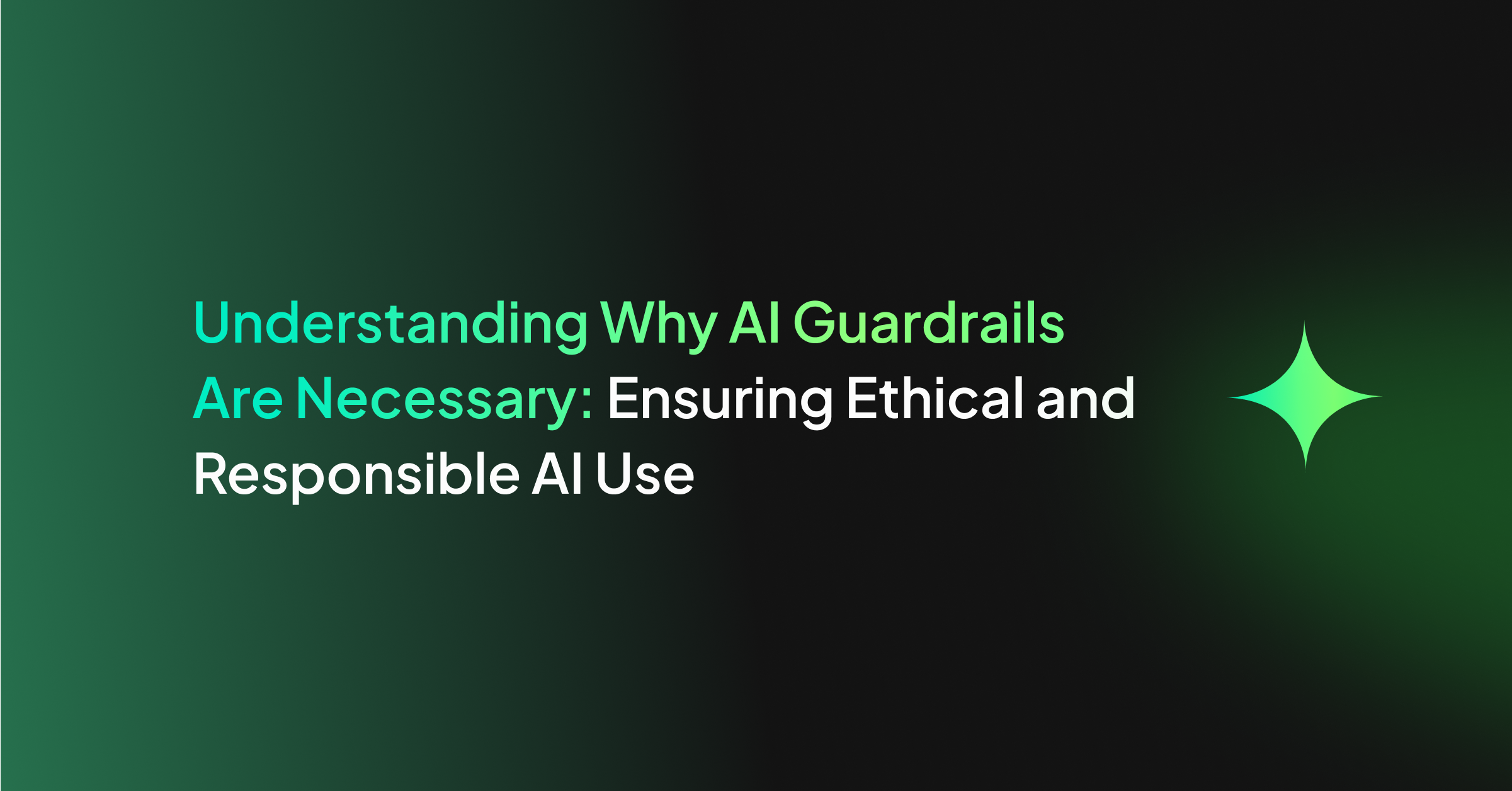
Artificial Intelligence (AI) has made tremendous strides in recent years, transforming industries and making our lives easier. But despite these advancements, expanding their use cases and impacting a wider range of areas, AI remains prone to significant errors. The promise of large language models (LLMs) is undeniable, offering impressive capabilities and versatility.
However, the risk of hallucinations and other generative AI errors continues to threaten user experience and brand reputation. These inherent performance risks underscore the persistent challenges in deploying AI effectively and reliably.
Let’s explore the concept of AI guardrails, their types, and their crucial role in ensuring AI apps are deployed safely, ethically, and reliably.
What are AI Guardrails?
AI guardrails are policies and frameworks designed to ensure that LLMs operate within ethical, legal, and technical boundaries. These guardrails are essential to prevent AI from causing harm, making biased decisions, or being misused. Think of them as safety measures that keep AI on the right track, like highway guardrails, which prevent vehicles from veering off course.
Isn’t Prompt Engineering Enough?
Prompt engineering, which involves designing and refining the backend prompts given to AI models, is a crucial aspect of AI development. However, relying solely on prompt engineering is not sufficient to mitigate hallucinations, where AI generates false or misleading information that often occurs with AI.
As more and more guidelines are added to the backend prompt, the LLM’s ability to follow instructions accurately rapidly degrades. Therefore, prompt engineering isn’t enough for engineers working to deploy reliable apps.
Does RAG Not Solve Hallucinations?
Retrieval-Augmented Generation (RAG) connects the LLM to a vector database allowing the LLM to provide results based mostly on the data provided, and not on the LLM internal knowledge. While RAG can improve accuracy and relevance, it does not entirely solve the problem of hallucinations. AI guardrails are necessary to detect and mitigate such issues, ensuring AI outputs are reliable and trustworthy.
For example, Air Canada’s chatbot gave a passenger bad advice by promising a discount that wasn’t actually available. The airline was forced to pay the price as a result. So even with prompt engineering and RAG, the system could still produce fabricated or inaccurate information, leading to misinformation. AI guardrails act as an external observer, ensuring that the results received by the AI system are accurate and legit.
Types of AI Guardrails
AI guardrails can be categorized into three main types:
- Ethical guardrails that ensure the LLM responses are aligned with human values and societal norms. Bias and discrimination based on gender, race, or age are some things ethical guardrails check against.
- Security guardrails that ensure the app complies with laws and regulations. Handling personal data and protecting individuals’ rights also fall under these guardrails.
- Technical guardrails protect the app against attempts of prompt injections often carried out by hackers or users trying to reveal sensitive information. These guardrails also safeguard the app against hallucinations.
3 Key Roles of AI Guardrails in Mitigating Risks in Generative AI
- Guarding Against Bias & Hallucinations
AI systems can inadvertently perpetuate or even amplify biases present in training data. AI guardrails help identify and correct these biases, ensuring that generative AI produces fair and unbiased content. Additionally, guardrails help detect and prevent hallucinations, ensuring the generated content is accurate and trustworthy.
A notable example is the use of AI in hiring processes. AI tools that analyze resumes and conduct interviews can introduce biases if they are trained on biased data. Implementing AI guardrails ensures these systems are regularly audited for fairness and adjusted to eliminate bias.
- Ensuring Privacy and Data Protection
Generative AI often requires access to vast amounts of data, raising concerns about privacy and data protection. AI guardrails ensure compliance with data protection laws and implement measures to safeguard personal information. This includes techniques such as data anonymization and secure data handling practices.
For instance, AI systems used in healthcare must comply with HIPAA regulations to protect patient data. Guardrails ensure that AI applications in this field do not compromise patient privacy.
- Preventing Misuse of AI
AI guardrails help prevent the misuse of generative AI for malicious purposes, such as by influencing the bot to say certain things. By implementing robust monitoring and control mechanisms, guardrails can detect and mitigate harmful activities, ensuring AI is used responsibly and ethically.
A real-life example is the use of an AI bot on a car dealership website. A user may trick the application to give it a wrong answer and then use this to ruin the brand’s reputation. Such as what happened with Chevrolet’s chatbot that agreed to sell a Chevy Tahoe for $1.
3 Common Challenges with Building AI Guardrails Solutions
While the importance of AI guardrails is clear, implementing them poses some challenges. These challenges can be categorized into technical, operational, and legal and regulatory.
- Technical Challenges
Implementing technical guardrails requires advanced engineering and robust testing. Ensuring that AI systems can handle edge cases and unexpected inputs without failing is a significant technical challenge. Additionally, developing methods to detect and mitigate biases and hallucinations in AI models requires continuous research and innovation.
- Operational Challenges
Operationalizing AI guardrails involves integrating them into existing workflows and systems. This requires collaboration across different teams, including data scientists, engineers, and legal experts. Ensuring all stakeholders understand and adhere to the guardrails is a critical operational challenge.
- Legal and Regulatory Challenges
Navigating the complex landscape of laws and regulations governing AI is a daunting task. Ensuring compliance with diverse legal frameworks across different jurisdictions requires significant effort and expertise. Additionally, as AI technology evolves, keeping up with changing regulations and adapting guardrails is a continuous challenge.