AIOps: Use Cases, How It Works & Critical Best Practices
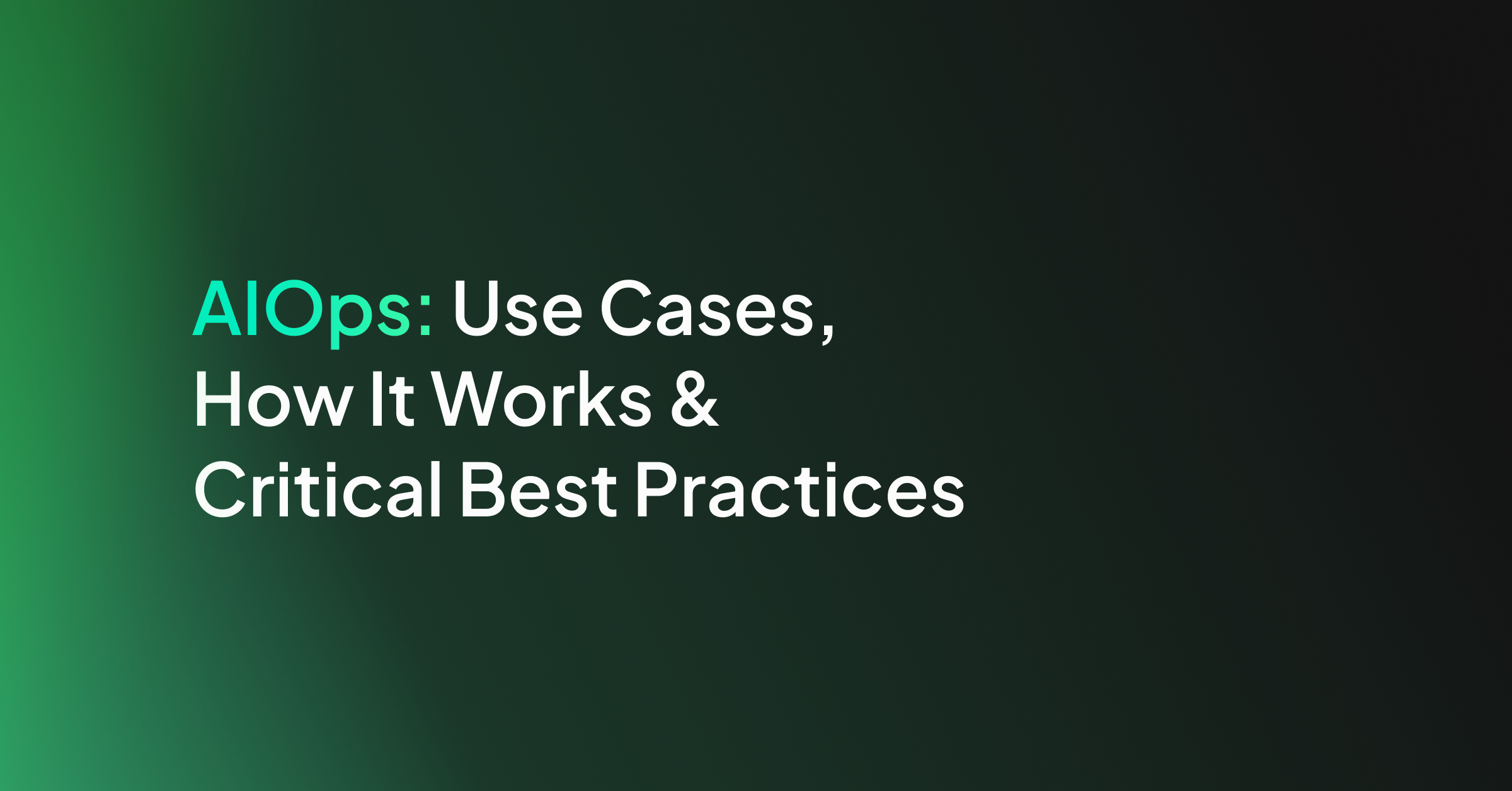
Common AIOps Use Cases
AIOps processes are suitable for a range of applications.
Root Cause Analysis
By analyzing vast amounts of data and uncovering dependencies between systems, AIOps determines the precise source of an issue, allowing IT teams to implement solutions promptly. Machine learning models aid in pattern recognition, which is crucial for tracing incident origins amidst complex infrastructures.
Anomaly Detection
Anomaly detection in AIOps involves identifying deviations from expected patterns in IT systems, indicating potential faults or security threats. Machine learning models in AIOps learn from historical data to establish what’s normal and flag anomalies in real time. Prompt detection allows IT teams to address issues before they escalate.
Performance Monitoring
By continuously analyzing metrics and logs, AIOps platforms track resource distribution, application health, and network performance. This surveillance helps maintain performance standards by allowing quick identification and correction of inefficiencies. AIOps also provides insights through dashboards and reports, enabling IT teams to make informed decisions regarding enhancements and capacity planning.
Cloud Automation and Optimization
By automating and orchestrating cloud-native resources, AIOps eliminates unnecessary manual configuration and monitoring, reducing complexity. Key metrics and performance indicators are analyzed to ensure optimal consumption and cost-effectiveness of cloud services. AIOps aids in dynamically scaling resources to meet demand changes, reducing waste.
Application Development Support
AIOps supports application development by fostering faster deployment cycles and increased efficiency. By integrating with DevOps practices, it offers real-time insights and analytics to developers, improving debugging times and throughput. AIOps also improves the reliability of CI/CD pipelines through automated testing, incident prediction, and anomaly detection.
AIOps vs Traditional IT Operations
Traditional IT operations rely heavily on manual processes and static rules-based systems to monitor and manage infrastructure. IT teams often work with siloed tools, leading to fragmented data analysis and slower response times. When issues arise, they typically follow a reactive approach, addressing incidents only after they have impacted systems or users.
AIOps integrates artificial intelligence, machine learning, and automation to shift IT operations from reactive to proactive management. Through real-time data analysis and predictive capabilities, AIOps can detect potential issues before they impact business functions. Instead of relying on isolated tools, AIOps platforms aggregate data from multiple sources into a unified system, providing a more complete view of the IT landscape.
How Does AIOps Work?
AIOps involves a structured, multi-step process that leverages data collection, machine learning, and automation to enable intelligent IT operations management. Here’s a breakdown of how it functions:
- Data ingestion and integration: The AIOps process begins with aggregating data from a range of sources, such as logs, metrics, traces, network data, and event alerts. This raw data, coming from applications, infrastructure, and network components, is ingested and then unified in a centralized repository. By standardizing and structuring data, AIOps creates a consolidated foundation for further analysis.
- Real-time monitoring and correlation: AIOps systems continuously monitor incoming data to identify patterns and anomalies. Using AI-driven correlation techniques, the system links related events across different sources, establishing a holistic view of IT operations. If several minor issues are detected on related infrastructure components, AIOps can recognize these as symptoms of a broader problem.
- Anomaly detection and pattern recognition: Once data is integrated, AIOps uses machine learning models to detect deviations from normal operational behavior, known as anomalies. Pattern recognition helps distinguish between typical fluctuations and signs of potential issues, enabling proactive response.
- Predictive insights and forecasting: With insights from historical and real-time data, AIOps applies predictive analytics to anticipate potential system failures, performance degradation, or capacity shortages. These forecasts help IT teams allocate resources more effectively, scale infrastructure based on demand, and preemptively resolve issues before they impact end-users.
- Automation and remediation: When issues are identified, AIOps triggers automated workflows to address them. For example, if an anomaly indicates a possible server overload, AIOps might initiate automated scaling or resource reallocation. By defining rules and leveraging machine learning, AIOps platforms can carry out actions like restarting services, rerouting traffic, or notifying relevant teams without manual intervention.
- Continuous learning and adaptation: AIOps can learn from incidents and continuously refine its functionality. As more data is processed, machine learning models improve, allowing AIOps to adapt to new patterns and changing infrastructure. This enables the system to respond to new scenarios.
Benefits of Implementing AIOps
Organizations can benefit from AIOps in the following ways:
- Faster mean time to repair (MTTR): By preventing incidents through early detection, AIOps ensures smoother IT operations. When incidents do occur, AIOps prioritizes and provides context-driven insights, accelerating root cause analysis and resolution.
- Reduced operational costs: By automating routine and labor-intensive tasks, AIOps decreases the need for manual interventions, freeing up IT resources to concentrate on strategic initiatives. This improves resource utilization and productivity across IT departments while cutting down operational expenses.
- Enhanced observability and collaboration: AIOps consolidates data from various sources into a centralized platform, giving IT teams insights into system performance and health. This visibility allows teams to work collaboratively, using shared data and analytics to diagnose issues and prevent future incidents.
- Predictive IT operations management: By analyzing patterns and historical data, AIOps anticipates disruptions and advises on preventive measures. This proactive approach reduces unplanned downtime and optimizes system availability.
Related content: Read our guide to AI observability
AIOps vs MLOps: What Is the Difference?
AIOps focuses on enhancing IT operations by automating incident response, monitoring, and system analysis, ensuring IT environments remain stable and responsive. It’s designed to simplify operational workflows, reduce downtime, and prevent disruptions by continuously analyzing IT data to detect and resolve issues proactively.
MLOps focuses on managing the lifecycle of machine learning models, from development to deployment and ongoing maintenance. Its main goal is to enable the operation of ML models in production by automating tasks such as model versioning, retraining, and performance monitoring. MLOps establishes best practices for collaboration between data science and operations teams, keeping machine learning models reliable in real-world applications.
Related content: Read our guide to real user monitoring
Best Practices for Implementing AIOps
Here are some of the ways that organizations can ensure the most effective implementation of AIOps.
1. Define Clear Objectives and Metrics
Establish precise goals for what the organization aims to achieve with AIOps, such as reducing MTTR or enhancing system resilience. These objectives should be measurable, enabling effective tracking and assessment of AIOps performance. Metrics should cover operational, performance, and business aspects to ensure comprehensive coverage.
Clear objectives also guide tool selection and process alignment, ensuring the right capabilities are prioritized.
2. Choose Relevant Data Sources
To effectively implement AIOps, it’s crucial to identify and integrate data sources that align with the organization’s strategy. Relevant data sources include:
- System logs from servers, networks, and applications
- Data about incidents from ticketing systems
- Data about IT assets from Configuration Management Databases (CMDBs)
- Application performance metrics from APM tools
- Events from Security Information and Event Management (SIEM) systems.
Understanding the formats, uses, and locations of this data is essential for successful integration.
3. Maintain High Data Quality for AIOps
Maintaining high data quality standards is vital for AIOps effectiveness. Ensure that the data is accurate, complete, and timely. Implement data validation processes and regular audits to maintain integrity. High-quality data enables AIOps to provide reliable insights and supports informed decision-making.
Some methods to ensure high-quality data include data cleansing, data preparation, and data mapping. Organizations can also use data integrity management or governance tools to enforce data quality standards.
4. Focus on Data Security
Data security is a fundamental aspect of any AIOps strategy, and should be the top priority. Protect sensitive information by implementing strong encryption, access controls, and compliance with relevant regulations. Regular security assessments and updates are important to protect against potential threats.
Data security policies are also important. For example, organizations can set rules to implement data masking policies for all sensitive data before being processed by an AI model.
5. Monitor Workflows to Optimize the AIOps Strategy
Monitoring workflows is essential to optimize the AIOps strategy. Regularly assess the performance of automated processes and the accuracy of AI-driven insights. Use these evaluations to refine algorithms and improve system efficiency. Continuous monitoring ensures that AIOps adapts to evolving IT environments and maintains optimal performance.
6. Ensure Human Oversight
While AIOps automates many processes, maintaining sufficient human oversight is crucial. Human expertise is necessary to interpret complex scenarios, make strategic decisions, and handle exceptions that automated systems may not address.
A balanced approach that combines automation with human judgment leads to more effective IT operations. For example, automated notifications can be used to prompt human investigation when more complex issues are detected.
AIOps with Coralogix
Coralogix sets itself apart in observability with its modern architecture, enabling real-time insights into logs, metrics, and traces with built-in cost optimization. Coralogix’s straightforward pricing covers all its platform offerings including APM, RUM, SIEM, infrastructure monitoring and much more. With unparalleled support that features less than 1 minute response times and 1 hour resolution times, Coralogix is a leading choice for thousands of organizations across the globe.
See Additional Guides on Key AI Technology Topics
Together with our content partners, we have authored in-depth guides on several other topics that can also be useful as you explore the world of AI technology.
AI Cyber Security
Authored by Exabeam
- AI Cyber Security: Securing AI Systems Against Cyber Threats
- AI Regulations and LLM Regulations: Past, Present, and Future
- Artificial Intelligence (AI) vs. Machine Learning (ML): Key Differences and
Examples
Open Source AI
Authored by Instaclustr
- Top 10 open source databases: Detailed feature comparison
- Open source AI tools: Pros and cons, types, and top 10 projects
- Open source data platform: Architecture and top 10 tools to know
Vector Database
Authored by Instaclustr