Feature Importance in Python: A Practical Guide
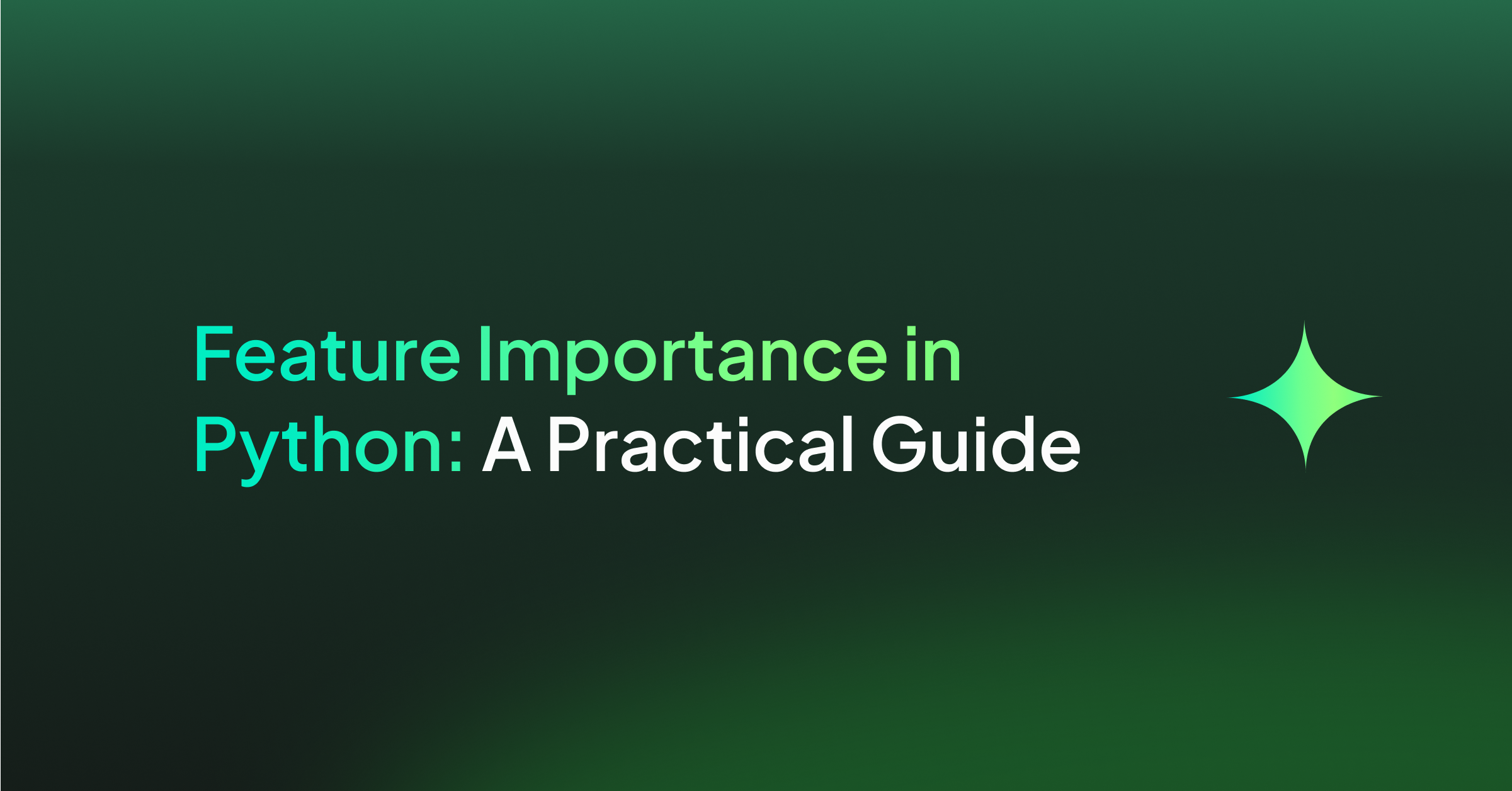
What Is Feature Importance?
Feature importance is a technique used in machine learning to determine the relative importance of each feature in a dataset when predicting a target variable. It helps to identify which features have the most significant impact on the model’s performance and can be used to reduce the dimensionality of the dataset by removing less important features.
Feature importance is typically measured using methods such as permutation importance, mean decrease impurity, or SHAP values. By understanding feature importance, data scientists can make more informed decisions about which features to include in their models and how to optimize them to improve overall performance.
Benefits of Using Python for Feature Importance
Using Python for feature importance provides several benefits due to its extensive ecosystem of libraries and tools, ease of use, and versatility. Some of the key benefits of using Python for feature importance include:
- Rich ecosystem: Python has a rich ecosystem of libraries, such as scikit-learn, pandas, NumPy, and XGBoost, which make it easy to implement various feature importance techniques. These libraries provide comprehensive functionality for data processing, machine learning, and statistical analysis, enabling seamless integration of feature importance computation into your data analysis pipeline.
- Flexibility: Python’s flexibility allows you to apply a wide range of feature importance techniques to different types of data and models. This enables you to choose the most appropriate method for your specific problem and dataset, ensuring the best possible results.
- Scalability: Python can be used for small-scale experiments and prototypes, as well as for large-scale production systems. This means that you can start by exploring feature importance on a small dataset and then easily scale up your analysis to handle larger, more complex datasets.
- Interoperability: Python’s interoperability with other programming languages and systems, such as C, C++, R, and Java, enables you to leverage existing code or libraries for your feature importance analysis. This can save time and effort, and allow you to integrate Python into your existing data analysis workflows.
Using scikit-learn for Feature Importance
Scikit-learn is a popular Python library used for machine learning and data analysis tasks. It provides a wide range of algorithms and tools for building predictive models, including methods for feature selection and ranking.
In scikit-learn, there are several ways to compute feature importance, including:
- Linear regression feature importance: Fit a LinearRegression model on the dataset and retrieve the
coeff_ property
that contains the coefficients for each input variable. These coefficients provide a crude feature importance score. - Logistic regression feature importance: Fit a LogisticRegression model on the dataset and retrieve the
coeff_ property
that contains the coefficients for each input variable. These coefficients provide a crude feature importance score. - Decision tree feature importance: Decision tree algorithms like CART offer importance scores based on the reduction in the criterion used to select split points, like Gini or entropy. This same approach can be used for ensembles of decision trees, such as random forest and stochastic gradient boosting algorithms.
- XGBoost feature importance: XGBoost provides an efficient implementation of the stochastic gradient boosting algorithm. After being fit, the model provides a
feature_importances_ property
that can be accessed to retrieve the relative importance scores for each input feature. - Permutation feature importance: This technique calculates relative importance scores that are independent of the model used. It involves fitting a model on the dataset, making predictions on a dataset with scrambled feature values, and repeating this process for each feature in the dataset. The result is a mean importance score for each input feature.
Feature Importance Example in scikit-learn
Here is an example of feature importance in Python that uses a forest of trees to calculate importance for artificial classification tasks. This example is based on the scikit-learn documentation.
The blue bars in the diagrams below show the forest’s feature importance alongside the inter-tree variability, represented as error bars. The plots used in this example suggest that only three features are informative but the rest are not. We can generate a plot using the following:
<span style="font-weight: 400;">import matplotlib.pyplot as plt</span>
Step 1: Generating Data and Fitting the Model
In this example we create a synthetic dataset of the three informative features. We’ll avoid shuffling the dataset so that these features correspond to the first three columns. We’ll also split the dataset into subsets for training and testing:
from sklearn.datasets import make_classification
from sklearn.model_selection import train_test_split
X, y = make_classification(
n_samples=1000,
n_features=10,
n_informative=3,
n_redundant=0,
n_repeated=0,
n_classes=2,
random_state=0,
shuffle=False,
)
X_train, X_test, y_train, y_test = train_test_split(X, y, stratify=y, random_state=42)
The next step is fitting a Random Forest classifier to calculate the importance:
from sklearn.ensemble import RandomForestClassifier
feature_names = [f"feature {i}" for i in range(X.shape[1])]
forest = RandomForestClassifier(random_state=0)
forest.fit(X_train, y_train)
Step 2: Calculating Importance Based on Impurity Decrease
Feature importances are provided by the feature_importances_ fitted attribute provides the feature importances, which are calculated as the standard deviation and mean of each tree’s decrease in impurity:
import time
import numpy as np
start_time = time.time()
importances = forest.feature_importances_
std = np.std([tree.feature_importances_ for tree in forest.estimators_], axis=0)
elapsed_time = time.time() - start_time
print(f"Elapsed time to calculate feature importances: {elapsed_time:.3f} seconds")
Next, we can plot the importance based on impurity:
import pandas as pd
forest_importances = pd.Series(importances, index=feature_names)
fig, ax = plt.subplots()
forest_importances.plot.bar(yerr=std, ax=ax)
ax.set_title("Feature importances with MDI")
ax.set_ylabel("Mean impurity decrease")
fig.tight_layout()
As expected, we can see that the first three features are shown to be important.
Step 3: Calculating Importance Using Feature Permutation
Permutation-based can help overcome the bias towards features with high cardinality but still relies on the relationship between features and the target variable. It is calculated on the training set, and can then be used to evaluate feature importance on the test set.
from sklearn.inspection import permutation_importance
start_time = time.time()
result = permutation_importance(
forest, X_test, y_test, n_repeats=10, random_state=42, n_jobs=2
)
elapsed_time = time.time() - start_time
print(f"Elapsed time to calculate feature importances: {elapsed_time:.3f} seconds")
forest_importances = pd.Series(result.importances_mean, index=feature_names)
However, this approach is more expensive because it shuffles features n times, refitting the model to estimate its importance:
fig, ax = plt.subplots()
forest_importances.plot.bar(yerr=result.importances_std, ax=ax)
ax.set_title("Feature importances with permutation on the full model")
ax.set_ylabel("Decrease in mean accuracy")
fig.tight_layout()
plt.show()
Both methods will identify the same features as the most important, although their relative importance may vary. However, the above plots reveal that mean decrease in impurity (MDI) is less likely to omit features than a permutation-based approach.