ML Observability Alternatives to Arize AI
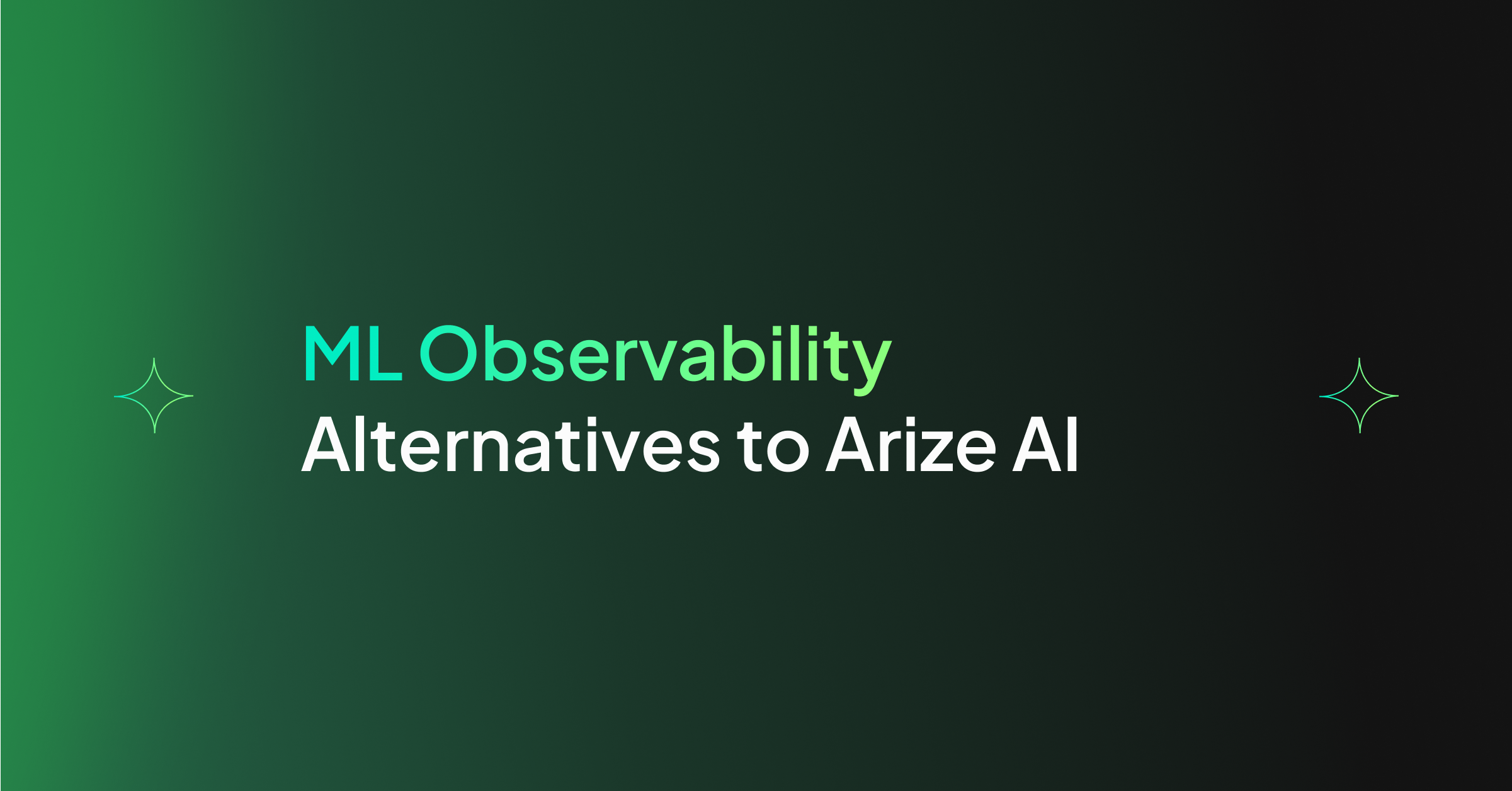
Looking for ML observability alternatives to Arize AI? Check out these solutions to help you get the most out of your ML models in production.
While Arize AI offers organizations a simple way to monitor ML models in production, it’s quite limited when it comes to providing actionable insights to improve model performance and investigate any issues in production.
In the ever-evolving landscape of AI/ML, businesses are continually seeking ways to improve and scale their production ML. As a result, several alternative solutions have emerged to help organizations streamline and optimize their ML processes. In this blog post, we will focus on 8 alternatives to Arize AI that are worth considering in 2024: Deepchecks, TruEra, Robust Intelligence, Superwise, SageMaker, Fiddler AI, WhyLabs, and Seldon Core.
1. Deepchecks
Deepchecks is an ML validation and monitoring platform that provides real-time insights into your models’ performance. The platform focuses on data validation, model validation, and monitoring, ensuring your ML models are accurate, reliable, and maintainable.
2. TruEra
TruEra offers a model intelligence platform designed to optimize the quality, explainability, and fairness of machine learning models. The platform helps data scientists and ML engineers understand and troubleshoot their models to improve performance.
3. Robust Intelligence
Robust Intelligence is an AI risk management platform that focuses on detecting and mitigating risks associated with AI deployments. It offers solutions to monitor, assess, and address issues like adversarial attacks, data drift, and model bias.
4. Superwise
Superwise is an AI assurance platform that provides visibility, control, and governance for AI applications. It enables organizations to ensure their AI models are trustworthy, reliable, and compliant with regulatory requirements.
5. SageMaker Model Monitor
Amazon SageMaker is a fully managed machine learning service that helps data scientists and developers build, train, and deploy ML models quickly and easily. SageMaker offers a wide range of tools and capabilities to support the entire ML lifecycle, from data preparation to monitoring and maintenance.
6. Fiddler AI
Fiddler AI is an explainable AI platform that helps businesses gain insights into their AI models, ensuring transparency, trustworthiness, and compliance. With Fiddler AI, organizations can improve their AI systems’ performance while adhering to regulatory and ethical standards.
7. WhyLabs
WhyLabs is a model observability platform that offers real-time monitoring and diagnostics for AI and ML applications. By providing a robust set of tools to detect data drift, identify anomalies, and troubleshoot issues, WhyLabs ensures that your ML models remain reliable and accurate over time.
8. Seldon Core
Seldon Core offers an ML monitoring solution for tracking and managing models in production. It provides real-time performance monitoring, alerts for deviations, and supports features like drift detection. Users can monitor metrics such as accuracy, latency, and throughput.
Conclusion
Each of these alternative solutions to Arize AI offers a unique approach to help businesses improve and scale their production ML. By understanding your organization’s specific needs and priorities, you can choose the right platform to optimize your ML processes and drive better results in 2025.