ML Model Monitoring: Practical guide to boosting model performance
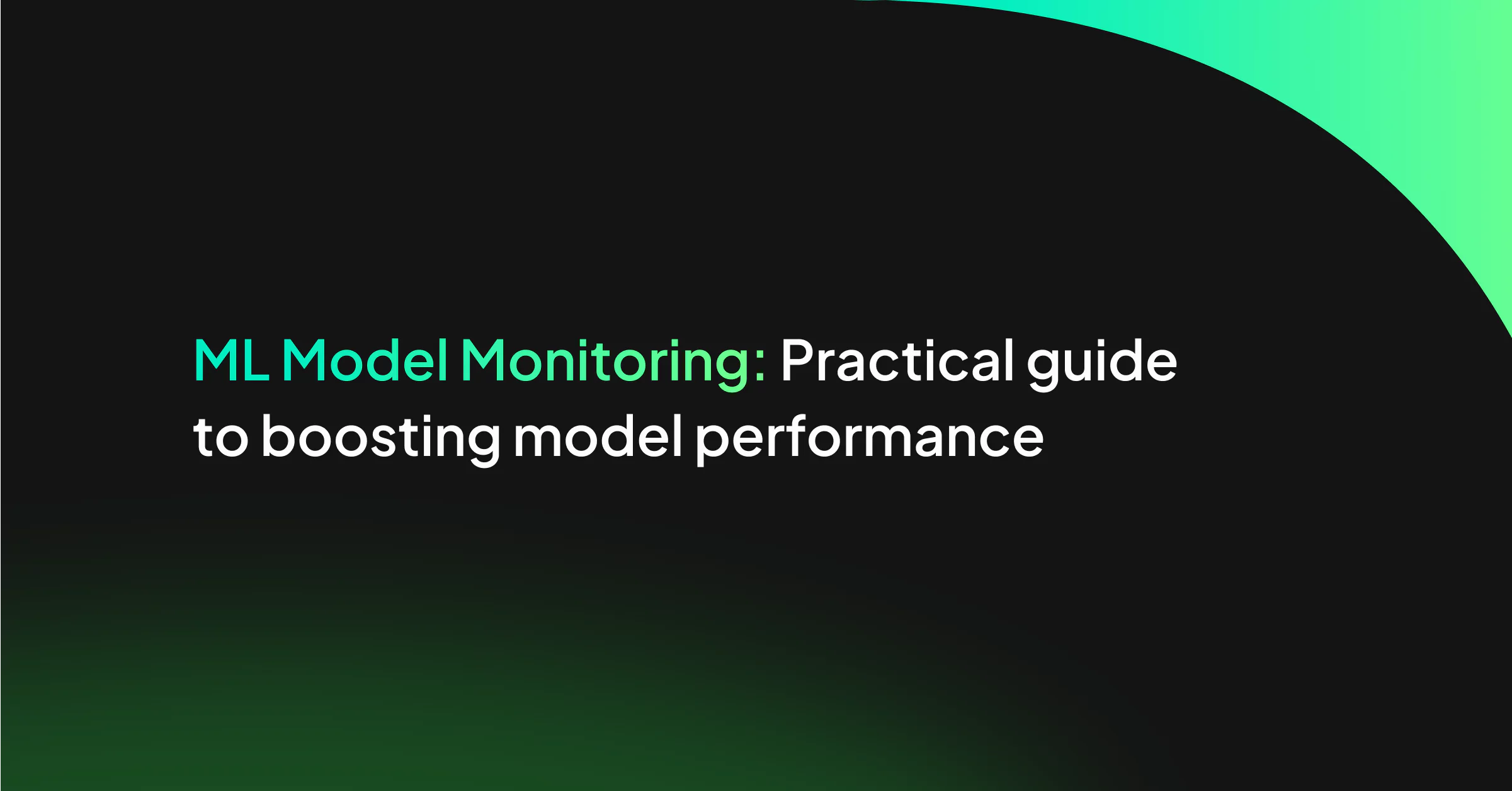
What is ML Model Monitoring?
Machine learning model monitoring measures of how well your machine learning model performs a task during training and in real-time deployment. As ML engineers, we define performance measures such as accuracy, F1 score, Recall, etc., which compare the predictions of a machine learning model with the known values of the dependent variable in a dataset.
Why ML Model Monitoring is Critical to Improve Performance?
When models are deployed to production, there is often a discrepancy between the original training data and dynamic data in the production environment. This causes the performance of a production model to degrade over time.
Machine learning model monitoring measures how well your machine learning models perform a task during training and in real-time deployment. As machine learning engineers and data scientists, we define performance metrics such as accuracy, F1 score, Recall, etc., which compare the predictions of machine learning models with the known values of the dependent variable in a dataset.
When machine learning models are deployed to production, there is often a discrepancy between the original data from model training and dynamic data in the production environment. This causes model performance to degrade over time.
For this reason, continuous tracking and monitoring of these performance metrics are critical for improving model performance. ML model monitoring can help by:
- Providing insights into model behavior and model performance in production.
- Alerting when issues arise e.g concept drift, data drift, or data quality issues.
- Providing actionable information to investigate and remediate these issues.
- Providing insights into why your model is making certain predictions and how to improve predictions.
These insights allow data scientists and ML teams to identify the root cause of problems, and make better decisions on how to evolve and update models to improve accuracy in production.
Why Should You Monitor Your Machine Learning Models?
1. Getting Feedback
In life, as well as in business, feedback loops are essential. The concept of feedback loops is simple: You produce something, measure how it performs, and then improve it. This is a constant process of model monitoring and improving. Machine learning models can certainly benefit from feedback loops if they contain measurable information and room for improvement. ML monitoring tools can provide that much-needed feedback.
2. Detecting Changes
Consider that you trained your model to detect credit card fraud based on pre-COVID user data. During a pandemic, credit card use and buying habits change. Such changes potentially expose your model to data from a distribution with which the model was not trained. This is an example of data drift, one of several sources of model degradation. Without ML monitoring, your model will output incorrect predictions with no warning signs, which will negatively impact your customers and your organization in the long run.
3. Mitigating AI Hallucinations
AI hallucinations can severely degrade the performance of models, particularly in high-stakes environments such as healthcare, where a single incorrect output could lead to a misdiagnosis with serious health implications. It’s essential to monitor and mitigate these hallucinations in LLMs to ensure the accuracy and safety of AI applications, maintaining user trust and reliability in decision-making processes.
4. Continuous Improvement of ML Models
Model building is usually an iterative process, so using a metric stack for model monitoring is crucial to perform continuous improvement as the feedback received from the deployed ML model can be funneled back to the model building stage. It’s essential to know how well your machine learning models perform over time. To do this, you’ll need ML model monitoring tools that effectively monitor the model performance metrics of everything from concept drift to how well your algorithm performs with new data.
ML Model Monitoring Checklist
Several steps are involved in a typical ML workflow, including data ingestion, preprocessing, model building, evaluation, and deployment. Feedback, however, is missing from this workflow.
A primary goal of ML monitoring is to provide this feedback loop, feeding data from the production environment into the model building phase. This allows the machine learning models to continuously improve themselves by either updating or using an existing model.
Here is a checklist you can use to monitor your ML models:
- Identify data distribution changes – when the model receives new data that is significantly different from the original training dataset, model performance can degrade. It is critical to get early warning of changes in the data distribution of model features and model predictions. This makes it possible to update the dataset and model.
- Identify training-serving skew – despite rigorous testing and validation during development, a model might not produce good results in production. This could be because of differences between the production and development environments. Try reproducing the production environment in training and if it performs better, this indicates a training-serving skew.
- Identify model or concept drift – when a model initially performs well in production but then degrades in performance over time, this indicates drift. ML monitoring tools or observability for machine learning systems can help you detect drift, track performance metrics, identify how it affects the model, and get actionable recommendations for improving it.
- Identify health issues in pipelines – in some cases, issues with models step from failures during automated steps in your pipeline. For example, a training or deployment process could fail unexpectedly. Model monitoring can help you add observability to your pipeline to quickly identify and resolve bugs and bottlenecks.
- Identify model performance issues – even successful models can fail to meet end-user expectations if they are too slow to respond. ML Monitoring tools can help you identify if a prediction service experiences high latency, and why different models have different latency. This can help you identify a need for better model environments or more compute resources.
- Identify data quality problems – model monitoring can help you ensure production data and training data come from the same source and are processed the same way. Data quality issues can arise when production data does not follow the expected format or has data integrity issues.
ML Monitoring and Optimization Techniques
How to Detect Model Drift
Detecting model drift involves monitoring the performance of a machine learning model over time to identify any degradation in its performance. This can be achieved through various methods:
- Track performance metrics: Regularly evaluate the model’s performance using appropriate metrics such as accuracy, precision, nDCG, recall, F1-score, or mean squared error, depending on the problem type (classification, regression, etc).
- Monitor data distribution: Analyze the input feature distributions over time and compare them with the training data distribution. Significant changes in the distributions may indicate data drift, which can contribute to model drift.
- Compare against a benchmark: Use a benchmark model, such as a simpler or more stable model, to compare the performance of the current model. If the primary model’s performance declines relative to the benchmark, it may suggest model drift.
- Monitor concept drift: Check for changes in the relationship between input features and the target variable. Techniques like statistical tests, hypothesis testing, or classifier-based approaches can help detect concept drift, which may lead to model drift.
- Perform model updates: Regularly retrain the model with new data to ensure it stays up-to-date with the latest data distribution. Drift can occur when there’s a significant difference in performance between the old and updated model.
Find out more about model drift in our blog ‘What is Model Drift and 5 Ways to Prevent It’.
How to Detect Data Drift
Data drift occurs due to changes in your input or source data. Therefore, to detect data drift, you must observe your model’s input data in production and compare that to your original data from the training phase. A strong indication your model is experiencing data drift can be noticed when training and production data don’t share the same format or distribution.
For example, in the case of changes in data format, consider that you trained a machine learning model for house price prediction. In production, ensure that the input matrix has the same columns as the data you used during training. Changes in the distribution of the source data relative to the original data will require statistical techniques to detect.
The following tests can be used to detect data drift and changes in the distribution of the input data:
- Kolmogorov-Smirnov (K-S) test – you can use the K-S test to compare the distribution of your training set to your inputs in production. The null hypothesis is rejected if the distributions aren’t the same, indicating data drift.
- Population stability Index (PSI) -the PSI of a random variable is a measure of change in the variable’s distribution over time. In the example of the house price prediction system, you can measure the PSI on features of interest, such as square footage or average neighborhood income, to observe how the distributions of those features are changing over time. Large changes may indicate data drift.
- Z-score – the z-core can compare the distribution of features between the training and production data. If the absolute value of the calculated z-score is high, you may be experiencing data drift.
How to Detect Concept Drift
You can detect concept drift by detecting changes in prediction probabilities given the input. Detecting changes in your model’s output given production inputs could indicate changes at a level of analysis where you are not operating.
For example, if your house price classification model is not accounting for inflation, your model will start underestimating house prices. You can also detect drift through ML monitoring tools and techniques, such as performance monitoring. Observing a change in the accuracy of your model or the classification confidence could indicate concept drift.
How to Prevent Concept Drift
Here are three ways to prevent concept drift:
- Model monitoring – reveals degradation in model performance that could indicate concept drift, thus prompting ML developers to update the model.
- Time-based approach – the ML model is periodically retrained given a degradation timeframe. For example, if model performance becomes unacceptable every four months, retrain every three months.
- Online learning –Performance monitoring helps us detect that a machine learning model in production is underperforming and understand why it is underperforming. Monitoring ML model performance often includes monitoring model activity, metric change, model staleness (or freshness), and performance degradation. The insights gained through model performance monitoring will advise changes to make to improve performance, such as hyperparameter tuning, transfer learning, model retraining, developing a new model, and more.Monitoring model performance depends on the model‘s task. An image classification model would use performance metrics such as accuracy and precision, but performance metrics like mean squared error (MSE) and mean absolute error (MAE) are better for a regression model.It is important to understand that a bad performance does not mean that model performance is degrading. For example, when using MSE, we can expect that sensitivity to outliers will decrease the model’s performance over a given batch. However, observing this degradation does not indicate that the model’s performance is getting worse. It is simply an artifact of having an outlier in the source data while using MSE as your metric.
To define what is considered poor performance in monitoring the performance of a machine learning model, we need to clearly define what is poor performance. This typically means specifying an accuracy score or error as the expected value and observing any deviation from the expected performance over time.
In practice, data scientists understand that a machine learning model will not perform as well on real-world data as the test data used during development. Additionally, real-world data is very likely to change over time. For these reasons, we can expect and tolerate some level of performance decay once the model is deployed. To this end, we use an upper and lower bound for the expected performance of the model. The data science team should carefully choose the parameters that define expected performance in collaboration with subject matter experts.
Performance decay has very different consequences depending on the use case. The level of performance decay acceptable thus depends on the application of the machine learning model. For example, we may tolerate a 3% accuracy decrease on an animal sound classification app, but a 3% accuracy decrease would be unacceptable for a brain tumor detection system.
the model trains every time new data is available, instead of waiting to accumulate a large dataset and then retraining the model.
How to Monitor ML Model Performance
Performance monitoring helps us detect that a production ML model is underperforming and understand why it is underperforming. Monitoring ML performance often includes monitoring model activity, metric change, model staleness (or freshness), and performance degradation. The insights gained through ML performance monitoring will advise changes to make to improve performance, such as hyperparameter tuning, transfer learning, model retraining, developing a new model, and more.
Monitoring performance depends on the model‘s task. An image classification model would use accuracy as the performance metric, but mean squared error (MSE) is better for a regression model.
It is important to understand that a bad performance does not mean that model performance is degrading. For example, when using MSE, we can expect that sensitivity to outliers will decrease the model’s performance over a given batch. However, observing this degradation does not indicate that the model’s performance is getting worse. It is simply an artifact of having an outlier in the input data while using MSE as your metric.
Defining what is considered poor performance
In monitoring the performance of an ML model, we need to clearly define what is poor performance. This typically means specifying an accuracy score or error as the expected value and observing any deviation from the expected performance over time.
In practice, data scientists understand that a model will not perform as well on real-world data as the test data used during development. Additionally, real-world data is very likely to change over time. For these reasons, we can expect and tolerate some level of performance decay once the model is deployed. To this end, we use an upper and lower bound for the expected performance of the model. The data science team should carefully choose the parameters that define expected performance in collaboration with subject matter experts.
Performance decay has very different consequences depending on the use case. The level of performance decay acceptable thus depends on the application of the model. For example, we may tolerate a 3% accuracy decrease on an animal sound classification app, but a 3% accuracy decrease would be unacceptable for a brain tumor detection system.
How to Improve ML Model Performance
Machine learning performance monitoring tools are valuable to detect when a production model is underperforming and what we can do to improve. To remediate issues in an underperforming model, it is helpful to:
- Keep data preprocessing and the ML model in separate modules – Keeping data preprocessing and the ML model as separate modules helps you fix a degrading model more efficiently when changes to the preprocessing pipeline are sufficient. Consider that you built a model that performs handwriting classification on mail in a US post office. In production, the post office decides to get lower intensity light bulbs for energy savings. Your model is now performing on much darker images. In this case, changing the data preprocessing module to increase pixel intensities and enhance borders is enough to improve model performance. It is also significantly less expensive and time-consuming than retraining the model.
- Use a baseline model – this is a simpler and more interpretable model that achieves good results. You use a baseline model as a sanity check for your big fancy production model. For example, the baseline for an LSTM for time-series data could be a logistic regression model. Observing a decrease in performance in your production model while the baseline model has good performance could indicate that your production model overfits the training data.
- Choose a model architecture that is easily retrainable – neural networks are powerful machine learning algorithms because of their ability to approximate any complex function. They are particularly well suited for production because it is possible only to train parts of a neural network. For example, when an image classification model encounters images from new classes, you can only retrain the classification part of the network with the additional classes and redeploy.
Key Capabilities of a Model Monitoring Solution
ML monitoring can be more effective with a dedicated monitoring solution. Look for the following features when selecting ML monitoring tools:
- Data drift detection – keeping track of the distribution of each input feature can help reveal changes in the input data over time. You can extend this tracking to joint distributions.
- Data integrity detection – to detect changes in the source data structure, check that feature names are the same as those in your training set. Scanning the input for missing values will reveal changes or issues in data gathering pipelines.
- Concept drift detection – knowing the importance of each feature in your input data relative to the output is a simple yet effective guard against concept drift. Variations in feature relevance are an indication of concept drift. These techniques also help understand changes in model performance.
Checking the input data establishes a short feedback loop to quickly detect when the production model starts underperforming.