Why EVERYONE Needs DataPrime
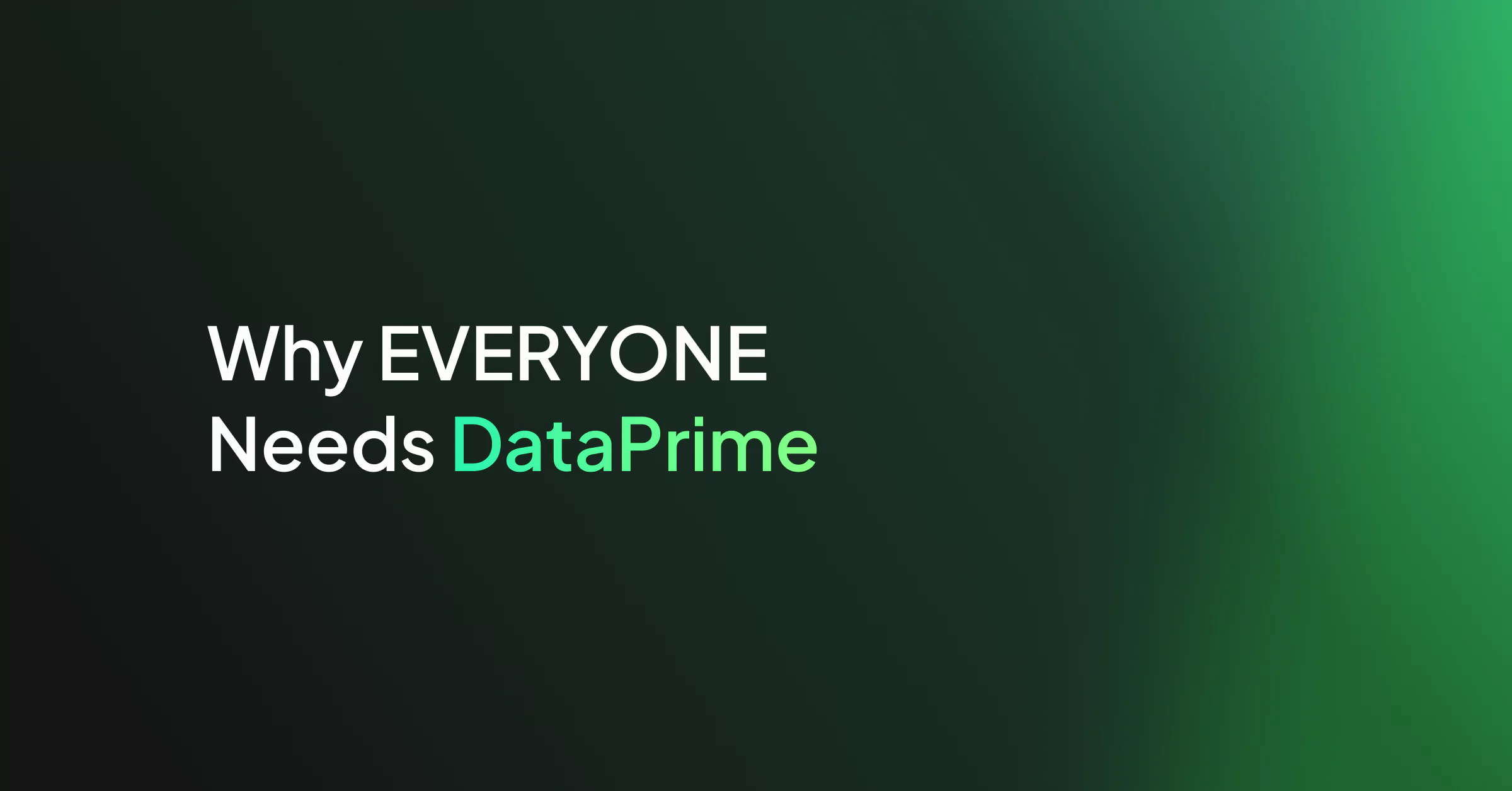
In modern observability, Lucene is the most commonly used language for log analysis. Lucene has earned its place as a query language. Still, as the industry demands change and the challenge of observability grows more difficult, Lucene’s limitations become more obvious.
How is Lucene limited?
Lucene is excellent for key value querying. For example, if I have a log with a field userId and I want to find all logs pertaining to the user Alex, then I can run a simple query: userId: Alex.
To understand Lucene limitations, ask a more advanced question: Who are the top 10 most active users on our site? Unfortunately, this is complex, requiring functionality that is not found in Lucene. So something new is necessary at this point. More than just a query language, observability needs a syntax that will help us explore new insights within our data.
DataPrime – The Full Stack Observability Syntax
DataPrime is the Coralogix query syntax that allows users to explore their data, perform schema on read transformations, group and aggregate fields, extract data, and much more. Let’s look at a few examples.
Aggregating Data – “Who are our Top 10 most active users?”
To answer a question like this, let’s break down our problem into stages:
- First, filter the data by logs that indicate “activity”
- Aggregate our data to count the logs
- Sort the results into descending order
- Limit the response to only the top 10
Most of these activities are completely impossible in Lucene, so let’s explore how they look in DataPrime:

DataPrime transforms this complex problem into a flattened series of processes, allowing users to think about their data as it transforms through their query rather than nesting and forming complex hierarchies of functionality.
Extracting Embedded Data – “How do we analyze unstructured strings?”
Extracting data in DataPrime is entirely trivial, using the extract command. This command allows users to transform unstructured data into parsed objects that are included as part of the schema (a capability known as schema on read). Extract supports a number of methods:
- JSON parsing will take unparsed JSON and add it to the schema of the document
- The key-value parser will automatically process key value pairs, using custom delimiters
- The Regex parser will allow users to define lookup groups to specify exactly where keys are in unstructured data.
The following example shows how simple it is to use regular expressions to capture multiple values from unstructured data.

Redacting – “We want to generate a report, but there’s sensitive data in here.”
Logs often contain personal information. A common solution to this problem is to extract the data, redact it in another tool and send the redacted version. All this does is copy personal data and increase the attack surface. Instead, use DataPrime to redact data as it’s queried.

This makes it impossible for data to leak out of the system, and helps companies analyze their data while maintaining data integrity and confidentiality.
DataPrime Changes how Customers Explore Their Data
With access to a much more sophisticated set of tools, users can explore and analyze their data like never before. Don’t settle for simple queries and complex syntax. Flatten your processing, and generate entirely new fields on the fly using DataPrime.