The Best AI Observability Tools in 2025
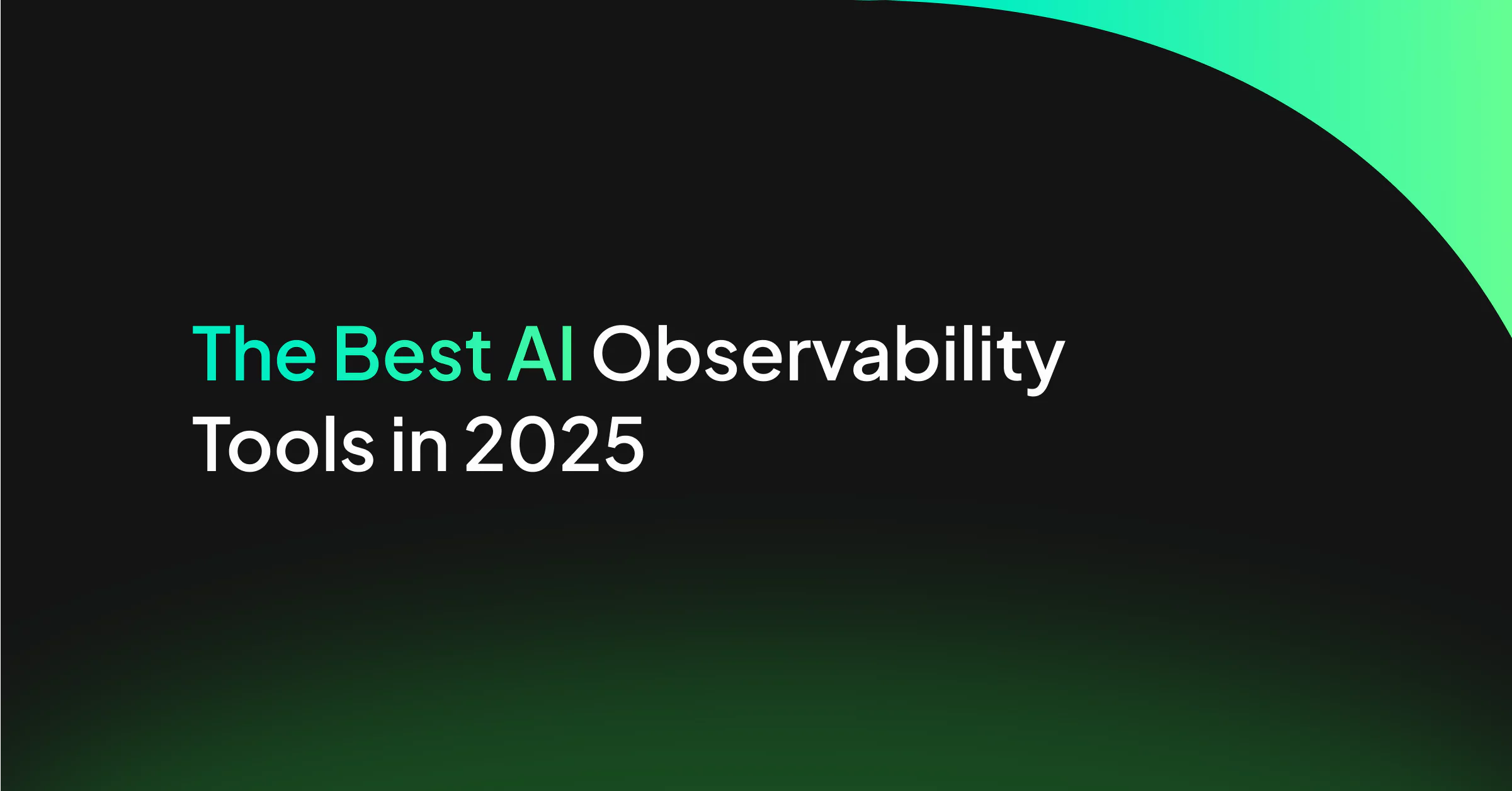
In 2025, AI isn’t just an add-on—it’s the engine powering everything from personalized customer experiences to mission-critical enterprise operations.
Modern systems generate 5–10 terabytes of telemetry data daily as they juggle intricate cloud-native architectures, microservices, and cutting-edge generative AI workloads. This sheer volume and complexity have pushed traditional monitoring to its limits, leaving a critical gap in proactive management.
Imagine having a panoramic view over your entire AI ecosystem—a real-time, unified dashboard that not only aggregates logs, metrics, and traces but also detects subtle anomalies before they evolve into costly disruptions.
AI observability tools provide precisely that: a holistic lens through which teams can continuously monitor, diagnose, and optimize the performance of their AI systems.
As the industry shifts from reactive troubleshooting to proactive management, these platforms are becoming indispensable for maintaining the high standards of reliability and security demanded by today’s digital enterprises.
In this article, we’ll explore the key features of AI observability platforms, closely examine some of the most robust tools on the market, and offer practical best practices to help you fortify your AI operations.
TL;DR
- Modern enterprise AI demands robust observability due to massive telemetry data, which is crucial for performance and security.
- Essential AI observability tools unify logs, metrics, and traces, enabling real-time insights and proactive anomaly detection.
- Key features include automated root cause analysis, customizable dashboards, AI-SPM, and specialized AI evaluators.
- Comprehensive solutions offer advantages through seamless integration, robust security, and user-journey tracking.
- Coralogix demonstrates the value of combining these capabilities for a holistic approach to AI system health and security.
Key Features of AI Observability Tools
The AI landscape is evolving rapidly, and there is a critical need for AI observability tools to eliminate guesswork and ensure seamless operations.
The observability platforms weave real-time monitoring, dynamic anomaly detection, and automated root cause analysis into a customizable interface that empowers teams to act before issues escalate.
Here are some key features of modern AI observability platforms.
1. Unified Metrics, Logs, and Traces
AI observability tools continuously pulse your entire cloud-native environment by collecting telemetry data—metrics, logs, and traces—specific to AI systems. Here’s how these elements relate to AI:
- Metrics: These include performance indicators like response times of AI models, token usage per request, or latency trends in generative AI pipelines.
- Logs: Logs capture detailed events such as user interactions with AI agents (e.g., prompts entered or responses generated), error messages from model inference layers, or API call details.
- Traces: Traces allow teams to follow the journey of a single user request through an AI system—tracking every step from input processing to model output generation.
For example, an AI observability platform could track all user actions within an AI agent application—monitoring latency spikes during peak usage or identifying errors when specific prompts cause failures.
2. Real-Time Monitoring and Data Aggregation
An always-on pulse continuously collects and aggregates telemetry data—metrics, logs, and traces—from every corner of your cloud-native environment. This constant data ingestion provides immediate, actionable insights into system health, empowering teams to detect performance degradation as it occurs and take action to prevent emerging issues.
3. Dynamic Anomaly Detection
Static thresholds simply can’t keep up with modern, elastic systems. Instead, AI observability tools use machine learning to learn what “normal” looks like and dynamically adjust baselines to detect subtle deviations.
Recent research on AI-driven anomaly detection demonstrated that deploying a solution across 25 teams reduced the mean time to detect (MTTD) by over 7 minutes—covering 63% of major incidents—translating to significantly fewer disruptions and improved uptime.
4. Automated Root Cause Analysis
Pinpointing their origin in a web of interdependent services can be daunting when anomalies occur. AI observability platforms automatically correlate data across multiple dimensions to identify the root cause quickly. This automated analysis accelerates troubleshooting and minimizes false positives, ensuring teams focus on critical issues.
5. Customizable Dashboards and Proactive Alerting
Customizable dashboards that seamlessly integrate with various cloud environments streamline the journey from insight to action. Tailored alerting mechanisms ensure that teams receive only the most contextually relevant notifications.
This proactive alerting eliminates unnecessary noise and aligns operational responses closely with business objectives, allowing teams to focus on what truly matters.
These features are not mere feature additions but strategic imperatives for modern enterprises. By reducing detection times, automating the correlation of diverse telemetry data, and delivering actionable insights, AI observability empowers enterprises to maintain high system reliability and drive continuous operational improvement.
6. AI-SPM (AI Security Posture Management)
AI Security Posture Management (AI-SPM) is a proactive strategy to secure AI systems at every stage of their lifecycle. It focuses on identifying, monitoring, and mitigating risks tied to AI technologies to ensure safe and compliant operations.
Key capabilities of AI-SPM include:
- Risk Assessment: Identifying vulnerabilities in training data, model behavior, or deployment environments to address potential threats early.
- Threat Detection: Monitoring for adversarial attacks, data breaches, or shadow AI usage that could compromise system integrity.
- Compliance Assurance: Ensuring AI systems meet regulatory standards like GDPR or NIST by continuously auditing data pipelines and model configurations.
7. AI Evaluators
Modern AI systems require specialized evaluators that go beyond traditional monitoring metrics. Evaluators are explicitly designed to identify common risks in generative AI applications:
- Hallucination detection: Identifying when models produce inaccurate or nonsensical outputs.
- Prompt injection monitoring: Detecting malicious inputs aimed at exploiting vulnerabilities.
- Toxicity scoring: Flagging harmful or inappropriate responses generated by models.
These evaluators ensure teams avoid risks unique to their AI systems while maintaining high-quality outputs.
8. User Journeys & Cost Tracking
Understanding user journeys through your AI systems is critical for optimizing performance and cost efficiency. It enables teams to visualize how users interact with their models—tracking steps like input processing times or response generation delays.
Additionally, cost-tracking features provide detailed insights into resource utilization:
- Token usage per request for LLMs.
- Compute costs associated with specific pipelines.
By combining user journey analytics with cost-tracking tools, the teams can effectively balance performance improvements with budgetary constraints.
Comparison of AI Observability Tools
The following table provides a side-by-side comparison of key features, ease of integration, and support for cloud-native/multi-cloud environments across the selected AI observability tools:
How to Read This Table:
OOTB and custom Evaluators
- Yes – Offers built-in (out-of-the-box) evaluators and supports creating custom ones.
- Partial – Provides some default evaluators but limited, complex, or no custom evaluator support.
- No – Does not have built-in evaluators or cannot create custom ones.
User Journey Tracking
- Yes – Enables complete, end-to-end tracking of all user interactions in real-time.
- Partial – Tracks only certain parts of the user journey or lacks continuous real-time visibility.
- No – Lacks integrated user journey tracking features.
The other columns (e.g., Simple Integration, Vendor Lock-In, AI-SPM, and Pricing) use Yes, Partial, or No similarly, indicating full, limited, or no support for the feature listed.
Tool | Connect via Open Source | Vendor Lock-In | OOTB and custom Evaluators | User Journey Tracking | Simple Integration | AI-SPM (Security Posture Management) | Pricing |
Coralogix | Yes | No | Yes | Yes | Yes | Yes | Simple, transparent pricing per tokens and evaluator usage |
New Relic | Yes | Yes | Partial | Partial | Yes | No | Expensive. Usage-based. Free tier available. |
Datadog | Yes | Yes | Partial | No | Partial | No | Modular, complex pricing per product. |
Dynatrace | Yes | Yes | Partial | No | Partial | No | Consumption-based, enterprise pricing |
EdenAI | Partial | No | Partial | No | Yes | No | Pay-per-use, no upfront fees. |
ServiceNow Cloud Observability | Yes | No | Partial | No | Yes | No | Rates not publicized. |
LogAI (Salesforce) | Yes | No | No | No | No | No | Open source |
Table 1: Comparison of different AI observability tools (By author)
Overall, these tools offer diverse approaches to AI observability. Organizations should evaluate factors like integration with existing workflows, scalability across cloud environments, and customizability.
If you are looking for a solution that effectively balances proactive issue detection with universal compatibility, carefully reviewing these options will help identify the best fit for your ecosystem.
Top AI Observability Tools
As AI-powered applications evolve, robust observability becomes critical. Traditional monitoring tools may capture generic data, but they often miss the nuances of AI agents—such as user interaction patterns and cost dynamics—without interfering in real-time operations.
1. Coralogix AI Observability
Coralogix AI redefines observability by offering actionable insights into all data types through seamless integration with OpenTelemetry. Unlike traditional monitoring tools that rely on static thresholds or limited compatibility with generative AI frameworks, Coralogix delivers real-time dashboards tailored to modern AI needs.
Key Observability Capabilities
- Real-Time Dashboards: Monitor every aspect of your AI systems in real-time—tracking errors, token usage, costs, and response times without disrupting user-LLM interactions. These dashboards provide instant visibility into user journeys to identify high-cost users or potential abuse scenarios.
- AI Evaluator Catalog & Custom Evals: Organize AI initiatives for targeted monitoring by the project. Build upon a library of specialized evaluators (e.g., prompt injection, hallucination, toxicity) to detect threats, data leaks, or malicious user behavior. Coralogix AI can even scan GitHub repositories to reveal where generative AI is used across the organization, helping minimize security exposure.
- Span-Level Tracing & Analytics: Zoom in on individual spans to pinpoint the root causes of errors, slowdowns, or anomalies. Compare app-specific latency metrics with organizational benchmarks for a broader context. In addition to standard performance data, a dedicated AI Security Posture Management (AI-SPM) dashboard provides deep insights into prompt injections, suspicious user activity, and potential cost-harvesting risks—alerting teams to denial-of-service threats or costly misuse.
- Risk Assessment & Metadata: At a glance, track the risk profile of each AI interaction, including user queries and LLM outputs. Coralogix AI highlights abusive behaviors and potential data leakage—whether accidental or malicious—so teams can respond proactively. While dashboards are not fully customizable and multimodal analysis isn’t currently supported, the platform’s single-click evaluator setup makes it easy for security officers to detect threats across all applications.
Coralogix AI goes beyond basic chatbot analytics, offering a dedicated, end-to-end observability product that addresses performance and security in a single interface. By centralizing real-time usage insights, risk assessments, and compliance checks, Coralogix empowers organizations to scale their AI systems confidently.
2. New Relic
New Relic’s Intelligent Observability Platform redefines AI monitoring by combining compound AI (multiple specialized models) and agentic AI (autonomous workflows) to predict system anomalies, automate root cause analysis, and link technical performance to business outcomes.
Designed for enterprises scaling generative AI, its core mission is democratizing observability through natural language querying and proactive issue resolution across hybrid cloud environments.
Key Observability Capabilities
- AI-Driven Anomaly Detection: The platform’s AI Engine analyzes logs, traces, and metrics in real-time, using machine learning to flag deviations in LLM token usage, API latency, and infrastructure bottlenecks.
- GitHub Copilot Integration: Collaborates with AI coding assistants to evaluate code changes pre-deployment, reducing incident risks from frequent updates.
- Natural Language to NRQL: Converts plain English queries into New Relic Query Language, enabling non-technical users to generate dashboards or trace AI pipeline performance without coding.
- Business Observability: Pathpoint Plus correlates IT metrics with KPIs like user engagement, offering no-code journey modeling and ML-enhanced incident insights for cross-functional teams.
While the platform excels in automating complex monitoring tasks and bridging technical-business gaps, its reliance on integrated ecosystems (e.g., GitHub, AWS) may require upfront configuration for teams without existing toolchain alignment.
3. ServiceNow Cloud Observability
ServiceNow Cloud Observability addresses AI system complexity through unified telemetry analysis, combining metrics, logs, and traces into a single platform powered by OpenTelemetry standards.
It streamlines incident resolution in distributed environments by automating dependency mapping and providing real-time visibility into cloud-native and legacy systems.
Key Observability Capabilities
- OpenTelemetry Integration: Maintains vendor-neutral data collection across hybrid environments and integrates with major cloud providers (AWS, GCP, Azure) and Kubernetes via OpenTelemetry.
- Unified Query Language (UQL): Enables cross-analysis of metrics, logs, and traces using a single syntax, with operators for filtering by attributes like customer ID or cloud region.
- AI-Powered Service Mapping: Automatically discovers and visualizes dependencies between microservices, enabling rapid root cause diagnosis for issues such as slow database queries through integrated telemetry analysis.
- Investigative Notebooks: Allows collaborative troubleshooting with real-time query sharing and embedded visualizations, reducing manual log correlation efforts.
While the platform excels in unifying observability workflows and integrating with ServiceNow’s CMDB, its effectiveness depends on OpenTelemetry adoption and may require configuration for non-ServiceNow ecosystems.
4. Open Source Tools – LogAI by Salesforce
LogAI addresses AI observability through a unified OpenTelemetry-compatible framework, enabling standardized analysis of logs across diverse formats and platforms.
Developed as a research-first toolkit, its core mission is democratizing AI-driven log analytics by eliminating redundant preprocessing through modular workflows for clustering, anomaly detection, and summarization.
Key Observability Capabilities
- Automated Log Summarization: Uses deep learning to condense extensive log data, enabling faster data review and anomaly recognition.
- Anomaly Detection: Continuously monitors log patterns to detect deviations and trigger alerts in real-time, reducing the mean time to detect.
- Log Clustering: Groups similar log events, simplifying root cause analysis and facilitating efficient troubleshooting.
- OpenTelemetry Integration: Ensures broad compatibility with existing monitoring stacks, enhancing overall system observability.
LogAI excels in academic research and customizable deployments. But its reliance on Python/PyTorch requires MLops expertise for production scaling. Unlike commercial tools like Coralogix, it lacks native infrastructure monitoring or SaaS SLAs, necessitating self-managed Kubernetes deployments for enterprise use.
5. Datadog
DataDog adopts a unified approach to AI observability by consolidating metrics, logs, and traces into a single cloud-native platform. Leveraging machine learning—primarily through its Watchdog feature—DataDog continuously analyzes telemetry data to proactively detect anomalies and perform automated root cause analysis, thereby reducing downtime and improving system reliability.
Although its scalability and extensive integration capabilities are significant strengths, the platform’s complexity may challenge smaller organizations with limited resources. Moreover, Datadog requires vendor lock-in as it does not support open standard connections like OpenTelemetry.
Key Observability Capabilities
- Unified Data Ingestion: Seamlessly aggregates metrics, logs, and traces from various environments, ensuring comprehensive visibility.
- Proactive Anomaly Detection: Employs ML algorithms (Watchdog) to flag deviations early, reducing downtime.
- Customizable Dashboards: Offers flexible, user-tailored visualizations and alerting workflows to suit diverse operational needs.
- Explainable Insights: Provides transparent root cause analysis, enabling informed decision-making.
Best Practices in AI Observability
Implementing effective AI observability requires a strategic approach that combines proactive issue detection, transparent insights, and customizable strategies. Here are some actionable best practices that enhance operational outcomes:
- Establish Clear Objectives: Define specific, measurable, achievable, relevant, and time-bound (SMART) objectives for AI observability, focusing on risk mitigation, performance optimization, or compliance.
- Implement Comprehensive Data Management: Use open standards like OpenTelemetry to reduce costs and enhance compatibility. Optimize data retention policies and evaluate data value versus storage costs.
- Set Baseline Metrics and Continuous Monitoring: Establish key performance indicators (KPIs) for AI models, such as accuracy and precision. Implement real-time monitoring and alerting for threshold violations.
- Leverage AI-Powered Insights: To identify issues early, utilize AI for automated anomaly detection and predictive analytics. Employ intelligent root cause analysis for faster issue resolution.
- Integrate Observability into AI Strategy: Make observability a core component of your AI strategy to ensure responsible, ethical, and sustainable AI operations.
By applying these practices, you can reduce downtime and enhance othe overall system reliability of your AI applications.
Conclusion
The quest for the right AI observability tool goes beyond simply collecting metrics and logs. It’s about ensuring the entire AI ecosystem remains stable and secure, even as data volumes and complexities scale exponentially.
By unifying real-time monitoring, automated anomaly detection, and proactive alerting, modern observability platforms can significantly reduce mean time to detection (MTTD). Recent research showed that dynamic anomaly detection reduced MTTD by an average of seven minutes, impacting over 60% of major incidents.
Automated root cause analysis and customizable dashboards enable teams to respond swiftly to incidents and derive actionable insights from high-level data overviews. As you assess your options, consider innovative solutions like Coralogix AI and others featured here.
Choosing a platform aligned with your organization’s goals and workflows will pave the way for more reliable, scalable AI operations—encouraging long-term growth and resilience.
FAQ
What is AI observability, and why is it essential for enterprise-scale AI systems?
Which AI observability platform offers a unified interface for modern AI systems?
What is AI observability, and how does it differ from traditional monitoring?
Which key features should an AI observability platform offer?
Why is real-time insight crucial for detecting and resolving AI anomalies?
References
- https://www.dynatrace.com/news/blog/what-is-observability-2/
- https://arxiv.org/abs/2404.16887
- https://coralogix.com/guides/aiops/ai-observability/
- https://lumigo.io/blog/shaping-the-next-generation-of-ai-powered-observability/
- https://coralogix.com/solutions/log-monitoring/
- https://coralogix.com/case-studies/jago/
- https://coralogix.com/platform/logs/
- https://coralogix.com/platform/apm/
- https://coralogix.com/blog/introducing-mobile-real-user-monitoring-rum/
- https://itbrief.com.au/story/new-relic-unveils-intelligent-observability-platform-with-ai
- https://www.businesswire.com/news/home/20241031538016/en/New-Relic-Unveils-Industry%E2%80%99s-First-Intelligent-Observability-Platform
- https://newrelic.com/blog/how-to-relic/ai-in-observability
- https://newrelic.com/platform/ai-monitoring
- https://newrelic.com/blog/nerdlog/new-relic-ai-assistant
- https://www.servicenow.com/au/products/observability/what-is-opentelemetry.html
- https://www.servicenow.com/au/products/uql.html
- https://www.servicenow.com/content/dam/servicenow-assets/public/en-us/doc-type/resource-center/data-sheet/ds-cloud-observability.pdf
- https://www.constellationr.com/blog-news/hot-take-servicenow-cloud-observability-knowledge23
- https://senser.tech/roi-of-observability-open-source-vs-commercial/
- https://censius.ai/blogs/oss-vs-commercial-tools-for-model-monitoring
- https://www.decube.io/post/open-source-data-observability
- https://www.cjco.com.au/article/news/unveiling-logai-revolutionizing-log-analysis-and-intelligence-for-academia-and-industry/
- https://ar5iv.labs.arxiv.org/html/2301.13415